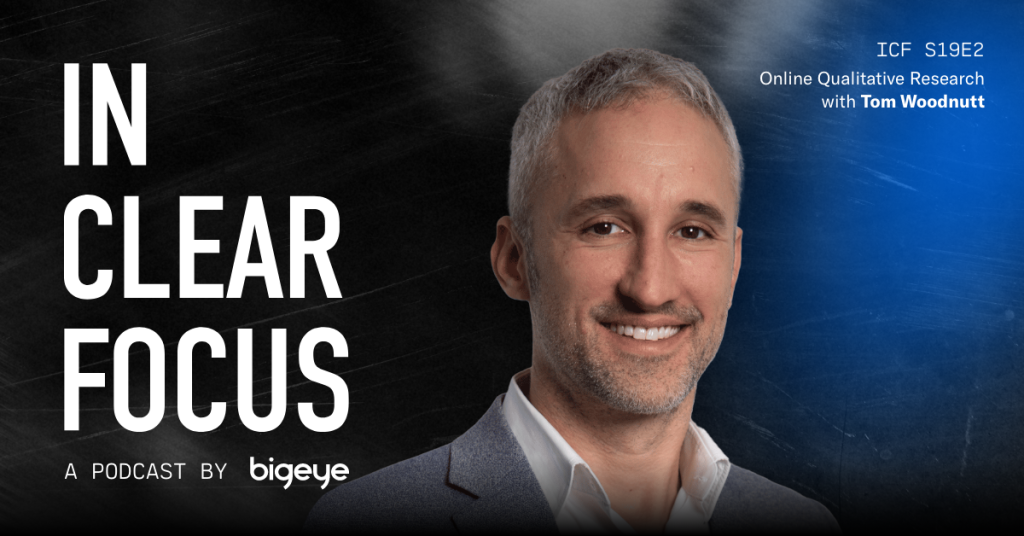
IN CLEAR FOCUS: Tom Woodnutt of Feeling Mutual discusses the evolution of online qualitative research. Tom explains how asynchronous methods yield authentic insights by allowing participants to share in real-world contexts without the social pressure of focus groups. He explores maintaining engagement, AI’s impact on research, and the “qual at scale” trend. While embracing AI as a powerful tool, Tom emphasizes the irreplaceable human intuition that makes great qualitative research possible.
Episode Transcript
Adrian Tennant: Coming up in this episode of IN CLEAR FOCUS:
Tom Woodnutt: I think ultimately great qual is about inspiring people to share authentic feelings and thoughts without inhibition. And I found that online tools allow you to do that in a more authentic, more open way than traditional methods.
Adrian Tennant: You’re listening to IN CLEAR FOCUS, fresh perspectives on marketing and advertising, produced weekly by Bigeye: a strategy-led, full-service creative agency growing brands for clients globally. Hello, I’m your host, Adrian Tennant, Chief Strategy Officer. Thank you for joining us. In today’s market research, the interplay between technology and human insight is reshaping how we understand consumers and markets. From digital qualitative research methods to the emergence of AI-powered analysis tools, researchers are navigating new opportunities and new challenges in gathering and interpreting consumer insights. Our guest today is at the forefront of these developments. Tom Woodnutt is the founder of Feeling Mutual, an award-winning insight consultancy specialising in online and mobile qualitative research. Tom has been pioneering digital qualitative methods since 2007, developing innovative approaches that combine the depth of traditional research with the authenticity and efficiency of digital tools. Tom has been nominated for the inaugural Wendy Gordon Pioneer Award in recognition of this. Tom speaks regularly at industry conferences and trains researchers to enhance their online qualitative skills. His work spans various sectors, including technology, media, CPG, and retail, collaborating with clients such as Amazon, Google, and UEFA. To discuss the evolution of online qualitative research, I’m delighted that Tom is joining us today from near Salisbury in England. Tom, welcome to IN CLEAR FOCUS.
Tom Woodnutt: Hi Adrian, great to be here.
Adrian Tennant: Well, you’ve worked in qualitative research for over 20 years and were an early adopter of digital methods. So what initially drew you to online qualitative research?
Tom Woodnutt: I think the seed was sown from having studied psychology at university, where I learned a lot about human unconscious biases. And so after a couple of years of moderating face-to-face groups as part of the job as a qual researcher I couldn’t help but get that feeling there was something artificial quite awkward and unnatural about those types of exchanges because ultimately you’re with this group of strangers you’re all sat around a table It’s a formal viewing facility, there’s cameras, microphones, a one-way mirror, clients are watching and they’re hyper aware of all of this and they can feel the political pressure that you’re under as well. And they’re very tuned into each other. And I just couldn’t shake off the knowledge that, as social animals, we can’t help but posture in groups and get influenced by what others say. And, you know, if someone annoys you, then you might just disagree with them for the sake of it. I’m sure we’ve all done it. If someone’s attractive, you might just agree. And so there’s also the fact that people will struggle to remember what they’ve done when you say, tell me what you did in a certain situation. You know, we’re not very good witnesses of our own behaviour when it’s out of context. So with the rise of Web 2.0 in the mid-2000s, having studied the psychology of computer-mediated communication as well, I started to really believe there was an opportunity in using blogging software and mobile video to get insight into people in a more intimate way. without the influence of others and more in the moment in real-world contexts. So you get insights just as people have had an experience in the real world. And don’t get me wrong, I do see value in face-to-face group discussions, and they do have some unique benefits over online methods. But overall, I’ve found that you can get much more depth, greater authenticity, and richer color from speaking with people one-on-one in their natural environment via online text and mobile methods. And I should clarify, I’m not really talking about Zoom-style focus groups as they suffer very similar threats to their authenticity and they can also inhibit emotional disclosure. I’m talking about using bespoke online qual tools that enable text feedback as well as mobile video collages and various other tools. The first time I experimented with this was back in 2007. And this was a project that I did for Innocent Drinks. And my friend Hugh Carling, who actually went on to found LiveMinds, which was one of the leading online qual platforms, he adapted WordPress software for us. And he was able to manually convert the videos that people took from the Innocent Village Fete, which was a kind of brand experience event. But you know, each video was taking an hour to upload. So this really was the old school days before the tech was there to do it in a more automated way as you have now. But what struck me in that project was that one of the most powerful outputs the client said they got was actually from the work we did, where we had a video of a family and a little girl dancing, which they thought perfectly captured the spirit of the event. And that was something that was shared broadly around the organization. And it was way more powerful, according to them, than any verbatim they could have got or did get from the focus groups that they also ran there. And that really struck me. And then from there, I moved into a role as digital director at my old agency, Hall & Partners, and my role was dedicated to developing and promoting online qual methods across the organization and the different offices. But then, since going solo 15 years ago, this is really something that I focused on: these types of online qual methods. And I’ve run lots of training presented at conferences with case studies and obviously using them for clients as well. Because I think ultimately for me, great qual is fundamentally about inspiring people to share authentic feelings and thoughts without inhibition. And I found that online tools allow you to do that in a more authentic, more open way than traditional methods.
Adrian Tennant: Tom, how has your research approach evolved over time?
Tom Woodnutt: Yeah, I think it’s changed with the times. So there have been three main changes to my method, which have reflected big changes in the world around us. The first one being Web 2.0, social media, and mobile, which all kicked off in the mid-2000s. And so this enabled what’s often called auto-ethnographic approaches, where people will share videos, photos, screenshots, text, whatever it is from a real-world context, live as it happens, without the interference of a researcher or any other participants being there. And so this means that we get details that they might forget if we were asking them to remember it as you do in focus groups, because we’re capturing it in the moment. And we find that people are very good at talking evocatively when they’re writing online or doing videos online. And sometimes you get, I would say, a more articulate response from people than you might do in the cut and thrust of a group discussion, for example. And so this basically means, because you’re speaking to people one-on-one, it means you’re getting way more depth from each person. So, for example, I might give someone an incentive that gets three hours of input from them across a week, say, whereas in a focus group to get two hours from them, you’re actually only getting 15 minutes from them because of the fact that only one person can speak at once. So it’s very much diluted in a focus group. Whereas online, they’re all doing it in parallel. So you really are getting more input for your incentive, and that results in a lot more depth, more than 10 times as much depth for the same number of people. The second big change was the rise of agile software development principles. Again, I guess around the 2010s, really, and a bit before then. So this is the idea I’m sure lots of listeners are aware of, iterating ideas rapidly – testing, developing them by building basic versions and then improving them based on how they perform. And this has been a big inspiration for the way that we use online qual methods, in particular, applied to the world of digital innovation, but also increasingly used in brand and communications research. One of my longtime clients, Fran Walton, who’s Head of Futures at Publicis Sapient, a big digital innovation consultancy, kind of developed this together in a way because he’s got his teams and colleagues who are designing rapid prototypes and ideas. And there isn’t much time to go from developing a strategy, getting feedback, developing creative ideas, executional ideas, and then testing that. So we’ve ended up compressing it into one piece of field work. And that requires a slightly different way of reporting. For example, a traditional qual timeline might be a case of testing some strategy ideas or going out and exploring the context to build those strategy ideas, going away, doing some creative development, then coming back and testing that creative development. And that’s a very linear process and takes a while. Whereas our approach, we combine it. So across a six-day study, the first few days are strategic exploration into the category, the context, and so on. And then we’re feeding back every day into a Mural or Miro, whatever it is, the best insights from each day so that the team can then develop the concepts that they’ve already developed the draft versions of. They can iterate them, improve them, and then we put them in front of people on the last couple of days of the field work. And then again, they can get the feedback from that and improve those ideas further. So essentially it’s crunching a strategic exploration and creative executional ideas development all into one. And I’ve since applied that to lots of advertising, strategy, and creative development briefs for the likes of Amazon and others. And it just means you can work in a much more agile way. You can fine-tune what you’re doing. And it just is much more suited to the timelines that we’re all under these days. So that’s another one. And then the third big change is obviously AI. Broadly speaking, it’s obviously opening up a lot of new methods, ways of doing things differently, at a greater scale, faster, cheaper, and so on. And it does represent a trade-off. There’s a trade-off over nuance and depth, but then, equally, that’s not necessarily always a priority in every brief. So it’s an interesting challenge and change to the way we do qual, but certainly, overall, I see it as an advantage, potentially, in some circumstances. But I always try and emphasize that trade-off that it represents.
Adrian Tennant: So, Tom, what is that trade-off?
Tom Woodnutt: I think the more you disintermediate the relationship and the proximity between the researcher and the participant, because say you’ve got auto-moderation or you’ve got AI summary tools, the less close we are to the data as a researcher, then some of the magic is lost and the confidence in conclusions are lost. Because often it’s when we’re moderating, we hear someone say something, we don’t know why, but it just intuitively, instinctively feels important, and feels insightful, and feels like it could inspire creativity for the client or smart decision making. That’s something that you’re less likely to experience if you’re not the one doing the moderation. If you’re just looking at a summary tool, typing in, “Tell me this …” “Who said that?” you’re missing out a whole load of data that you’re not aware of because you haven’t done the moderation. And so I think it comes at a cost. So if you’re disintermediating that relationship, then you’re also losing a bit of the confidence in the conclusions. Because researchers are quite cautious lot. We like to know that we’ve done our due diligence, we’ve read the transcripts, we know what was said, we’re confident in our version of the truth that we’re coming with. And as soon as you’re relying on the technology to get you to those conclusions and you’re not so close, then I think you lose a lot of confidence and therefore validity as well.
Adrian Tennant: That’s a really interesting point. Tom, in your experience, what are the key differences between synchronous online focus groups and asynchronous qualitative research methods?
Tom Woodnutt: So the main difference between online focus groups that are in real time or live, when you’ve got the likes of Zoom or whatever platform, and you’ve got six or so people for say 90 minutes, effectively just replicating the face-to-face group method. There are advantages in terms of you can see their faces, you can see their reactions to things. That said, it is probably more diluted than you would see when you’re in the room with them in person. So in that respect, it’s probably a weaker version than face-to-face, in my opinion. There’s an advantage in that because you’re there talking to them live, you can explain the stimulus, which sometimes can be complex. And also, clients can view live. I think that’s probably the biggest advantage over the asynchronous methods, because a client can hear firsthand, and sometimes that’s really important. But then again, there is this lack of depth because you’ve got to rush from person to person. You’ve only got 90 minutes. Only one person can speak at once. So there’s only so many probes you can ask. And also people get bored. I mean, we’ve all spent more time on Zoom calls than we’d probably like in recent years, and people switch off. They go on their phone, even when you tell them not to, sometimes. There’s issues there. Plus, it’s prone to all those other social force issues to do with groups where they might posture based on someone else that might be influenced by what someone else says. Ultimately, you run out of time, which might be okay if you don’t want to probe much, but I like to dig deep into what people are saying to really to go further. Whereas asynchronous methods – which is quite an annoying word that often means different things to different people – but generally we use that to highlight the fact that it’s running over a few days. So it’s not in real time. So we might do a question in the morning, and they might answer it in lunchtime, or others might answer it in the evening. But each day you’re getting that half an hour input from people, but yeah, it’s running over a few days. And the main benefits for me really is first of all, depth. I mean, you get so much more depth because that three hours of input you get from someone or two hours, whatever you’re paying them an incentive for, you get all of that time. They’re not sitting there waiting for someone else to finish speaking, but moreover, the authenticity. So again, the fact that you’re not influenced by others, the fact that you’re getting it in a natural context, a real-world context. That they’re remembering details they might have forgotten if they were having to remember back as they would be in a focus group method. You get the multimedia, the color, the video, the pictures they might upload. And then there’s that agility we talked about already, the idea that you can go back to people more easily, and you can speak to people all around the world. It’s very easy to do it internationally. And you’ve got these instant transcripts as well. So, I mean, yes, you can go and get transcripts. Often, people get AI transcripts, which aren’t perfect. But here, when people are writing a lot of the answers, you’ve got an instant set of words, which you can put straight into AI summary tools to support you. And yeah, of course, you’ve got that multimedia content, which makes reporting a lot more colorful and impactful, which is a key part of doing a good project.
Adrian Tennant: Well, you mentioned that online qual can generate significantly more input per participant than traditional focus groups, whether in person or online. How does this impact the depth and quality of insights?
Tom Woodnutt: Yeah, there is a logic to the more deeply you speak with someone, the more you probe their answers, the more space you give them to express themselves. The more you look at a topic from multiple angles, the more you’re going to learn. So overall, I think this extra depth you’re getting is a blessing because you get so much more rigor and detail. You can cover so much more ground, and it’s really important in digital innovation projects where there’s a lot of nuance and detail that really matters, the pain points. You might be testing quite a few different ideas, so you want that extra time with them. And so there’s also a blessing in the confidence it gives you in the findings. You’ve got all your transcripts there instantly, and often the quality of what they say, because they’ve typed it out, quite often is a bit more well articulated, well thought through, compared to the “um”s and “ah”s that we often get when we speak. But the main blessing for me is probably the emotional disclosure, because people are much more open. They’re not feeling so judged. They’re not aware of other people that are in the project because you can just set it to being private. And so you do get a lot more confessional insights, and often it’s those anxieties, those emotional dimensions of people’s relationships and experiences where the most useful insights are because they’re the ones that are harder for someone to assume or predict. That said, it can also be a bit of a curse because of that extra depth, you’ve got more volume of data to get through. Yes, AI can help, but it really isn’t doing the same thing as a human can do. And the more you rely on it, it comes at a cost. So ultimately, it does require more consultancy time to moderate and analyze – which is difficult if some clients assume that online is cheaper. And it’s not necessarily cheaper when you’ve got to do a proper job of analysis on it. But overall, I see the benefits certainly outweigh any drawbacks.
Adrian Tennant: Let’s take a short break. We’ll be right back after this message.
![]() | Alan Barker: Hello, I’m Alan Barker, the author of “The Complete Copywriter: The Definitive Guide to Marketing with Words,” published by Kogan Page. I’ll show you how to exercise your creativity, generate powerful ideas, maintain reader attention, and bring your copy to life. You’ll also learn how to develop a coherent content strategy, how to survive as a copywriter, and how to nurture a satisfying career. Whether you’re a professional writer already, a brand manager, or someone who creates content as part of another kind of job, this book will help you to develop the skills to craft compelling, customer-focused copy. As a listener to IN CLEAR FOCUS, you can save 25 percent on “The Complete Copywriter” when you order directly from Kogan Page. Just enter the exclusive promo code BIGEYE25 at the checkout. Shipping is always complimentary for customers in the US and UK. I hope my book helps you to become a more versatile, effective, and confident copywriter. Thank you! |
Adrian Tennant: Welcome back. I’m talking with Tom Woodnutt, founder of Feeling Mutual, about the evolution of online qualitative research. As regular listeners know, we love case studies on IN CLEAR FOCUS. So, Tom, can you explain how asynchronous online qualitative research can lead to authentic insights that we might not get from traditional focus groups?
Tom Woodnutt: Yeah, one of the main benefits, I think, is the privacy and the fact that you’re giving people a space to feed back in their own world, in their own comfortable environment without a researcher necessarily grilling them. And so one of the earliest examples of this was probably one of the first projects I did, where we were talking to mums about yogurt and the challenge of feeding kids healthy food. And we did focus groups, and we did the “online private blog space” as we called it back then. And what we noticed was when you got a group of mums – it applies to dads as well – talking about that challenge of feeding kids healthy food, they’re much less comfortable talking about it when there’s a group of other people there and they’re feeling a little bit judged potentially. Whereas when it’s private online, we had so much more articulation of really the nuance, the emotional challenges around it. So that’s a good example of getting that emotional disclosure. One of my favorite things that I ever got out of a piece of research was for Google Banking, where we were looking into the finance sector on behalf of Google. And we asked people to draw how they feel in terms of their relationship with their bank. And there was a drawing that someone did that was just brilliant of picture of a girl holding a teddy bear, standing in front of these giant, really imposing gates saying, “I feel completely powerless in the face of this giant faceless bank,” which really captured that power differential between banks and their customers in a way that then was, you know, a big part of the report. And it was really useful to them because a picture speaks a thousand words, as they say. Then we’ve done some video feedback for people around drinking and parties, and what role does alcohol play in parties and different brands. And so people were giving us footage of their actual party, which was quite fun. And also not the kind of thing you could necessarily just turn up as a researcher without ruining the vibe, as they say! Yeah, another one would be, again, in the context of a real experience, electric vehicles. We got people to talk about how they feel while they’re filling up their electric vehicle, and we got a sense of that, I guess, boredom, and the wait, and so on. And that was to inspire new ideas for gas stations in the US and how they could serve electric vehicles better and make the experience better. So again, something you wouldn’t have got unless you sent an ethnography team with a video camera there, which would also perhaps make them a bit self-conscious. Yeah, I think there’s lots of ways you get stuff that you get through that privacy, that intimacy that you have in this method.
Adrian Tennant: Well, Tom, you’ve talked about the importance of designing studies that keep participants motivated. What strategies have you found most effective for maintaining engagement in asynchronous research?
Tom Woodnutt: Yeah, I mean, first of all, there’s obviously our friend hard cash, which people respond well to! But you know, it’s really important that you’re honest about how long it’s going to take and be accurate. And so I’ll often, if ever it does feel like it’s going on more, I’ll pay people extra to reflect that because I think it’s important to respect their time. But beyond money, I mean, really, you don’t want to make it just about these kind of extrinsic incentives. You want to make them feel important, make them feel listened to, give them questions that are more interesting to take part in and to answer than just boring set of direct questioning. So it could be a creative exercise, like “Imagine you’re the marketing director …” or “Draw a picture of how you feel …” or “How would you spend this budget on if you could split between these things …?” Just something where it’s maybe role play or more interesting than your average direct question. Because I think it’s our responsibility as researchers to engage them and make them feel motivated. Because on day three, they may not come back. It’s not like a focus group where they’re stuck in the room and they’re unlikely just to walk out if they’re not having fun – although that did happen to me once! It’s really more, you know, you have to design it in a way that’s engaging and realistic and fair to them, and you’ve got to pay them, but also make them feel respected, and then hopefully they’ll open up. And also, actually, I find the warm-up, this is something that Wendy Gordon often talked about, and how important the warm-up is in core research. It’s not just a basic politeness thing, although there is that factor. It’s also, if you can let people articulate who they are and get their identity out, then they’re more likely to be themselves throughout. So I always ask questions at the beginning about “How would your best friend describe you?” “What’s a picture that sums up your values?” And get quite deep with people, because people like to talk meaningfully and authentically. And once they’ve done that, then the real selves, I think, are more likely to come out to play, as it were. So yeah, that’s a couple of examples.
Adrian Tennant: Wendy Gordon is really the doyenne of qualitative research in the UK.
Tom Woodnutt: Yeah, she was the biggest contributor to, I think, all the good things that qual has become.
Adrian Tennant: Tom, you recently spoke at the Market Research Society’s AI conference in London. What were some of the key themes that emerged about AI’s role in qual research?
Tom Woodnutt: It was a really interesting conference, I’ve got to say, but there was an interesting feeling in the room as well. There was almost a tension; there was this palpable excitement at the prospect of what AI can do and hearing all the really progressive examples of it in action. There’s also an anxiety, this idea that it might take over our job, which no one wants, obviously. And sometimes it does feel there is a conflict at the heart of it. Sometimes I call it The Uber Paradox, this idea that Uber drivers are essentially training the Uber business to be able to replace them eventually, as their stated aim is, with fully autonomous self-driving cars. And so there’s a sense that is that what we’re doing, the more we use AI tools, the more we’re helping it get better until the point comes when it just replaces us. And I’d like to think that that’s not the case. And I still think that humans have a pivotal role at the helm. But the conference was really excited with all the new innovations going on in the space of research and AI. And it’s unsurprising that generative AI is having such an impact on qual research in particular, because the currency of qual is language, and so is the currency of large language models that drive generative AI. So AI can process and find meaning in linguistic information. That’s exactly what we humans do as well. So it can inform ideas and study design. It can automate chatbot moderation, which enables qual at scale and at lower cost. It can automate the summarizing of texts, which means more data can be processed. And of course, there’s synthetic users as well, where the idea of proxies for real humans just based on algorithms predicting what people might have said. And obviously they don’t get tired and they don’t need incentives. So there’s lots of crossover. But to quote Michael Hoosman, he said that “Language is the Wild West in terms of data because it goes off in infinite directions. It’s not binary. There’s so much that is unspoken, subtle subtext, irony, meaning in the gaps in what people say. The meaning is more than just the words.” It’s very hard for an algorithmic prediction engine to perform like a brilliant human qual researcher. It could perform like an average one, don’t get me wrong, but it doesn’t know what we know. It doesn’t know what we know about the client, the craft, the strategy, or research, or the human condition. It doesn’t have that intuition and empathy. It takes everything at face value. And it tends towards the norm in its interpretations, which can lead to quite generic, bland outputs. So it really needs a human to humanize what it produces and to make it distinctive. Another difference, and we talked about this quite a lot at the conference, is that ultimately authenticity and originality of thought are the lifeblood of a great qual researcher but it’s certainly not how AI works. So AI could mechanize knowledge and information, but it can’t mechanize wisdom, and that’s what humans really bring. So I think the view on how much AI can disrupt qual really depends on how you view qual research because for me, a qual researcher is interpreting what they’re hearing or seeing and representing a single version of reality. They’re curating a version of the truth that’s authentic, it’s data-led. But it’s also very intuitive and it’s selective because we don’t put every single quote into the report. We have to choose the ones that are strategically valid and useful to the client. It’s not a science. It’s not an objective reality we’re sharing. It’s a craft. And there are multiple realities we could share, but we pick the version that’s most valuable. And that’s based on experience, intuition, judgment. And these are things that the AI doesn’t have. It’s a prediction engine. It just guesses the next word that seems sensible. and it says what it thinks might be plausible, or not what it thinks, but what its algorithms tell it is likely to be plausible based on the appearance of words in its learning data, and so on. It’s not as good at understanding intuitively what’s going to be inspiring and useful and strategically valid. It’s just a tool, but it’s a very powerful tool, and it’s only as good as the person using it. So I think we are going to see a rise in things like DIY qual, where non-experts are doing more qual research. But I still think it’s the trained experts who are best placed to do it better because we can be the gatekeepers of strategic work. We can judge authenticity. We know which verbatim are authentic. We know how to make insights that are novel, inspiring, and kind of turn that light on when they’re heard. So overall, I think, yeah, it’s a very exciting thing. There is some conflict there. There’s a lot of resistance as well from people. They feel very threatened by it, and they’re quite reluctant to try it. Or when they do try it, they’re looking at it as if “it’s me versus them,” rather than “me with AI and what I can do with it.” So I think that’s the kind of more constructive mindset that I’ve been trying to take as well. And yeah, interesting times and challenging times, but I am optimistic and I’d like to think that the more people are doing qual, even the non-experts, the more people will realize the true value of true expertise in qual and hopefully it can actually be a golden age for qual rather than a threat to it.
Adrian Tennant: That’s a very positive outlook. Your case study for PureGym demonstrates both traditional online qualitative methods and the innovative use of AI. So could you walk us through that project and what you call the second pressing of insights?
Tom Woodnutt: Yes, I like the way “traditional online qual” is together now. Obviously, we’ve reached that point where online is no longer this brave new frontier, which is refreshing.
Adrian Tennant: I thought you’d like that.
Tom Woodnutt: Yeah! But what we did was, it’s another example of combining strategic exploration and creative development. So for PureGym, they wanted to test some creative work. They also wanted to just scrutinize the strategy they had and understand the category, how people choose to get membership or not with the gym, how they see the competition, and also the cost of living, understanding how people were making decisions about what to keep, what to get rid of in terms of subscriptions and so on. So we spent the first three days exploring all that context and then that was fed back through to the agency. So they were able to iterate the scripts based on what we told them from those first few days. And then we tested the scripts and then we came back with our report fairly quickly so they could then in time for Christmas, get their ad campaign out, which went really well for them. They had a lot of growth in the subsequent months, but we kind of thought, it’s a bit of a shame that there’s all that exploration into the strategic context, all of that really great emotional stuff about being intimidated in gyms, touches on mortality, all these amazingly deep stuff that just wasn’t in the debrief because it was so focused on the creative work. So actually we said, look, a year on, why don’t we go back over all of that exploration data using AI to make it faster and quicker and ultimately affordable. And we’ll put together a report based on our prompting of the AI to pull out the story and to find the verbatim. And so they gave us a fresh brief that was pertinent for them all around converting the high awareness that developed through the ads and the successful campaign into actual membership. And we did that and it was really interesting to realize that actually a lot of these insights you get from people are kind of evergreen. They don’t just stop being relevant. Obviously, that doesn’t apply in all trend-based research, but there are some fundamental truths that you can find in one point and then go back to at a later point, they’re still really useful. And thanks to AI – and we use CoLoop, the analysis tool, – we got to that much faster and made it possible. But it should be noted also the fact that we had done the moderation and that we knew we had the confidence to use the tool and were able to use the tool better. It still shows that proximity can benefit and you can get more out of AI tools when you are close to the people.
Adrian Tennant: Because you’d moderated the sessions, you also knew what to prompt.
Tom Woodnutt: Yeah, definitely. I think it goes back to that point that if the qual researcher, often the hypotheses we develop happens in the field. The ideas we get for a great angle on reporting doesn’t just come as we’re reading transcripts. It happens while you’re asking questions. And so I think all of that knowledge we had from having asked the questions meant that we were able to know what we were looking for and therefore could get more value through the AI tool.
Adrian Tennant: So how do you see the relationship between human researchers and AI tools evolving over the next few years?
Tom Woodnutt: Well, the first thing that’s already happening is what they’re calling qual at scale and the idea that you can do quant sample sizes. So, you know, even hundreds of people, whatever. And you can ask them open-ended questions, they can be automatically probed, and you can automatically process the answers and get data out of it. So it’s a kind of more open version of quant in many ways. And that’s really how I see it. It’s an improvement on quant rather than an improvement on qual. Because for me, the whole basis of qual is you speak to less people but in more depth, you recruit them carefully, you design the study carefully so you can extrapolate what they say. and apply that to a bigger population. For me, qual at scale is one of the less exciting ones for the type of brief I work on. I’m sure there are briefs where it’s great. Inevitably, automated data collection at scale and automated thematic analysis is going to be growing. Also, interestingly, I think there’s going to be a lot more around things like emotional facial coding, integrating that into the types of conversations we have so that expressions are encoded and even biometric data analysis, I think there’s going to be a lot more inputs like that taken into account as well. And also I think making our reports more media-rich, so being able to get to faster video edits, films that embed the insights across the organization better because you’ve had the AI-assisted analysis and editing tools. And then of course there’s synthetic data where you’re just using AI to predict what someone might say in response to a question. And I think that’s really useful at the upfront stage of a study. So, you know, why not write your discussion guide, get a bunch of bots to answer it based on whoever the sample is. And then that can only help if you see that you’re like, “Ooh, there’s a hypothesis” and thoughts of “Why don’t we ask that question?” when we commit to the design. And it just gets you a headstart before you do the actual study. So I think that’s a useful application of it.
Adrian Tennant: Yeah, I love that idea of using the synthetic participants before you unleash your discussion guide on the real ones. Tom, what advice would you give to researchers who are just starting to incorporate AI tools into their qualitative research practice?
Tom Woodnutt: The number one thing is to experiment because you have to really try it to work out your way of using it, how it complements your style as a researcher, as a thinker. I think being an expert in your organisation will help and then you can share the learnings with others. communicate the shortcomings of it as well. You don’t have to oversell it, but I think get out of that mindset of it’s “me versus it,” as if it’s like, “oh, let’s just prove how humans are better. I’ll be safe, I’ll keep my job.” That’s an unhealthy way of looking at it. It’s better to think “This is here, this is happening. How can I use my unique skills to get more value from it than someone who didn’t have my skills?” And when you look at it in that constructive, collaborative, like it’s a co-intelligence rather than a threat, I think then you’re going to get the most value from it. And also just trying different tools, maybe going to clients and saying, “Hey, we’re going to do this demo. We’ll do it for cheap, help fund it. You can get to learn about how AI works as well.” And just trying to experiment is really the main thing. I guess if you’re talking about younger people coming into the industry, that does raise quite a big challenge, which I don’t know what the answer is to this. But I do think that if lots of new researchers who’ve just come into the industry, if they’re given a load of AI tools and told, you know, “Off you go!” I do wonder how they’re going to develop those skills of qual because they are often forged in the heat of the trenches when you’re doing your 65th group in Scunthorpe or wherever it is. So I think if you don’t have that hands-on experience, I do wonder what you lose because it’s quite a hard-fought set of skills, qual. because you have to go through a lot of conversations, sometimes about stuff that you’re not necessarily interested in, sometimes about things you are interested in, but it’s a long slog and the craft of writing a story, working out what’s important, articulating it, having the confidence to put your neck on the line with a view, all of these things take time to develop and I don’t know how you do that if you’re just given automatic tools, but hopefully, yeah, it’s something industry can talk about and we’ll find a way through that.
Adrian Tennant: I love your idea of AI as a co-intelligence with the human researcher. For listeners who want to learn more about your work at Feeling Mutual or to connect with you, what’s the best way to do so?
Tom Woodnutt: I think LinkedIn is the best place. You know, I put a bit of content out there. The website is another way to get in touch as well, feelingmutual.com. And yeah, I’d love to hear from anyone that’s interested in what we’ve been talking about. So yeah, drop me a line. And thank you also, yeah, and I would urge everyone to check out your other podcasts because there’s several I’ve been listening to and they’re really, really interesting stuff.
Adrian Tennant: Tom, thank you very much for being our guest this week on IN CLEAR FOCUS.
Tom Woodnutt: Absolute pleasure, thank you.
Adrian Tennant: Thanks again to my guest this week, Tom Woodnutt, founder of insights consultancy, Feeling Mutual. As always, you’ll find a complete transcript of our conversation with timestamps and links to the resources we discussed on the IN CLEAR FOCUS page at Bigeyeagency.com. Just select ‘Insights’ from the menu. Thank you for listening to IN CLEAR FOCUS, produced by Bigeye. I’ve been your host, Adrian Tennant. Until next week, goodbye.
TIMESTAMPS
00:00: Introduction to Online Qualitative Research
00:16: Welcome to IN CLEAR FOCUS
00:37: The Intersection of Technology and Human Insight
01:08: Introducing Tom Woodnutt
02:01: Tom’s Journey into Online Qualitative Research
05:52: Evolution of Research Approaches
06:03: Impact of Web 2.0 and Social Media
07:16: Agile Software Development Principles in Research
09:22: The Role of AI in Qualitative Research
10:57: Trade-offs in AI Integration
11:09: Synchronous vs. Asynchronous Research Methods
13:44: Depth and Quality of Insights in Online Qual
15:34: Case Studies in Authentic Insights
19:30: Maintaining Engagement in Asynchronous Research
21:18: Wendy Gordon’s Influence on Qualitative Research
21:30: AI’s Role in Qualitative Research
26:00: Case Study: PureGym and AI Integration
29:00: Future of Human Researchers and AI Tools
31:00: Advice for Researchers on AI Tools
32:52: Connecting with Tom Woodnutt
33:35: Conclusion and Thanks