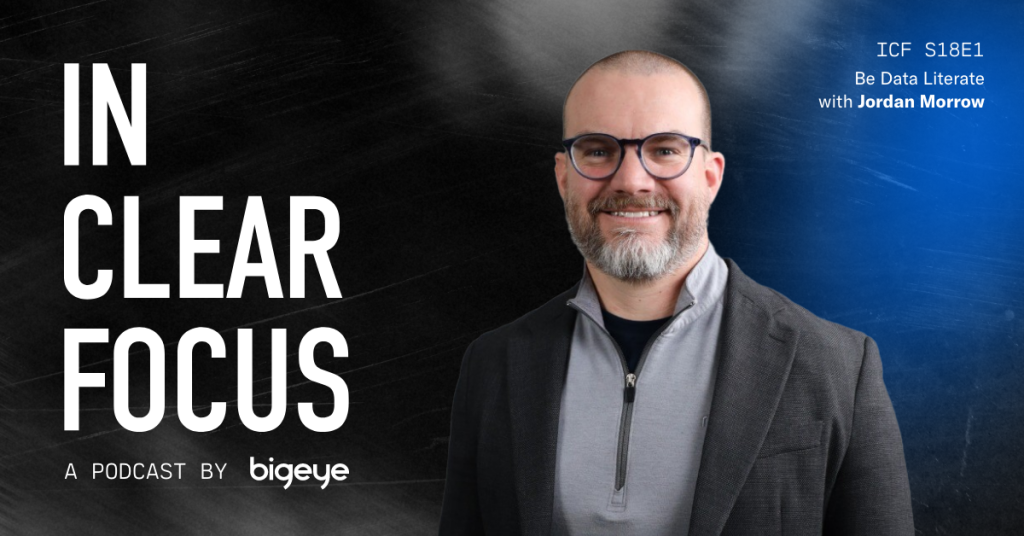
IN CLEAR FOCUS: Jordan Morrow, SVP of Data and AI Transformation at AgileOne and the “Godfather of data literacy,” discusses the intersection of AI and data literacy. Jordan shares insights on the four levels of analytics, the “three C’s” of data literacy, and practical steps for organizations to build data confidence. As the author of “Be Data Literate,” Jordan emphasizes that while not everyone needs to be a data scientist, understanding data is crucial in today’s AI-driven business world.
<
Episode Transcript
Adrian Tennant: Coming up in this episode of IN CLEAR FOCUS
Jordan Morrow: How do we tie AI with data literacy? As we watch the world continue to evolve at a pace that is unbelievable right now when it comes to AI and technology we need everybody to be confident and comfortable in using data to make decisions.
Adrian Tennant: You’re listening to the first episode of the eighteenth season of IN CLEAR FOCUS, fresh perspectives on marketing and advertising produced weekly by Bigeye, a strategy-led full-service creative agency growing brands for clients globally. Hello, I’m your host, Adrian Tennant, Chief Strategy Officer. Thank you for joining us. In today’s digital age, data has become the lifeblood of business decision-making. Yet, despite having access to more data than ever before, many organizations struggle to derive meaningful insights from their information. The challenge isn’t just about having data, it’s about understanding it, working with it effectively, and using it to make informed decisions. This raises an important question. In a world where not everyone needs to be a data scientist, how can professionals become more data literate? Our guest today is known as “the Godfather of data literacy,” having helped pioneer the field by building one of the world’s first data literacy programs. Jordan Morrow is the Senior Vice President of Data and AI Transformation at AgileOne and the founder and CEO of Bodhi Data. He previously served as the Chair of the Advisory Board for the Data Literacy Project and has helped organizations worldwide, including the United Nations, build and understand data literacy. Jordan is also the author of four books, including “Be Data Literate: The Data Literacy Skills Everyone Needs To Succeed,” which won the International Book Awards 2023 for Business Technology. Now in its second edition, the book has been updated to include insights into the future of data and artificial intelligence, And it’s also our Bigeye Book Club selection for this month. To discuss data literacy and its growing importance, I’m delighted that Jordan is joining us today from Bountiful, Utah. Jordan, welcome to IN CLEAR FOCUS.
Jordan Morrow: Well, thank you for having me. Thank you for letting me be a book of the month. This is fantastic, thank you.
Adrian Tennant: Well, Jordan, let’s start with the basics. What exactly is data literacy, and why is it becoming increasingly important in today’s business environment?
Jordan Morrow: Yeah, so real quick, just give a baseline definition: it’s the ability to read, work with, analyze, and communicate with data. So, there are four key characteristics that everybody can develop skills in. And I think it’s very important to make clear it’s not data science, right? We don’t need everybody to become super nerdy, super tech-oriented. We just want people to be able to develop those four key characteristics. Again, it’s reading, working with, analyzing, and communicating with data. Now, the big question comes in, why is it important? And it’s even more important with the advent of AI, without a doubt. This is why we wrote a second edition. The conversation with my publisher was, “Do we write a whole new book, or do we just add to this one?” And there’ll be plenty of books on AI. So how do we tie AI with data literacy? And as we watch the world continue to evolve at a pace that is unbelievable right now, when it comes to AI and technology, we need everybody to be confident and comfortable in using data to make decisions. And I’ll make one last statement on this is everybody already is data literate to a degree. We use our weather app when we determine the weather. I’m in the state of Utah, where we get snow, it’s cold, but I’m traveling next week. So I took a look at the weather next week, and I’m gonna know from that to make a decision. That’s data literacy. And so I think far too often, people think of data literacy in a more technical sense. In reality, we’re all data literate. Can we help you now get more comfortable using data and AI to make decisions in a business setting?
Adrian Tennant: In your book, you outline four levels of analytics. Jordan, could you walk us through these and explain why understanding them is crucial?
Jordan Morrow: Absolutely. So there are four levels of analytics, and I’m not sure how many people know that, especially if you’re not like a statistician or a data scientist analyst. So, the four levels are descriptive, diagnostic, predictive, and prescriptive analytics. Now I’m going to use an analogy to walk people through what these analytics are. I want everybody who listens to this, just imagine that you’re sick, right? Not very fun to imagine that you’re not feeling well. But when you’re sick, what do you do? You go to a doctor and that doctor says to you, “You’re sick,” and leaves the room. How many people would stay visiting that doctor, right? If that’s all they did was tell you that you’re sick, “Here’s your illness,” move on. You’re probably not going to go back to them. But what that doctor did was gave you a descriptive analytic. They described the situation. And in the business world, it’s the same thing. How many dashboards do we see all the time? How many KPIs? How many metrics? Well, those things are important. It’s important for the doctor to let you know your illness. Absolutely. But in the business world, we’ve got to go beyond what is happening to why it is happening. And that is your diagnostic analytics. So same thing with the doctor. Doctor says “You’re sick. Here is why.” Because then once they know why, they will make a prediction on what you can do to get better. And sometimes they gave you a prescription. And you’ll see all of these analytical levels are a part of that scenario. And it’s the same thing in a business setting. We have dashboards in analytics. Well, we need to go beyond descriptive analytics. We need to figure out why those things are occurring. So, we get our diagnostic analytic. Once we have that, we can start to build predictions to see where things may go if we make decisions. And then finally, sometimes we let the machine learning and the technology do it, which is a prescriptive analytic. These matter greatly, and organizations should be focused on bringing all four of these to the organization. I want to make something very, very clear. In an organization of, say, 10,000 people, you’re going to have maybe 50 to 100 who are data professionals by trade and title. That means 9,900 or more of those who are not data professionals. So where do you think they’d spend most of the time? Well, it’s going to be in descriptive and diagnostic analytics. building dashboards, using dashboards, trying to figure out why things are happening so that they can turn the more technical analytics over to the data professionals. It’s not a divide these in four, so 25 percent of your time is spent in each. Majority of a business’s time is spent in descriptive and diagnostic. And we build predictions and prescriptions around things. Now, one final note on these four levels of analytics is that it is a recurring thing. How many of you have ever been misdiagnosed by a doctor, right? They don’t get it right every time. Neither does analytics. Even if something is 95% likely to happen, five times out of 100, it’s not going to happen based on the probability. So when we tie it to data literacy, I don’t need everybody building predictive analytics. I don’t need everybody building prescriptive analytics. But we want to get you comfortable talking about them maybe and getting more familiar with descriptive and diagnostic analytics.
Adrian Tennant: The second edition of “Be Data Literate” came out in September 2024. What prompted you to update the book, and what major changes did you make?
Jordan Morrow: Well, it all revolved around AI. In fact, we paused me writing my fourth book so we could get a second edition of book one out. The big change is AI. Now, again, the way that I write is I’m not writing AI from a technical spot. I’m not writing machine learning and teaching people how to be engineers. When it comes to data literacy in this topic of AI, it’s very similar in the sense, can we empower people to be more confident in how they’re using AI to effectively make decisions in their job? That’s really what this boils down to. It’s not major changes to the original. It’s call it an evolution and an addition to the original in that we brought AI to the table. And for 90 to 99% of people who read that book or hear about AI and things, I’m going to boil it down to three characteristics: Can you prompt the AI well? Can you evaluate the prompt response it gives you? And then can you execute a decision upon it? There’s a lot of buzz and a lot of things around AI right now. For someone like me, that’s my day-to-day job. I need to dive in and understand it more than most. But for the majority of the people, it’s finding good use cases on how to apply this new AI technology to what they do. So with the second edition, bring AI to the data literacy world, bring AI to people’s minds, hopefully still delivering a message that you don’t have to be an advanced technical person with AI unless you want to be, right? So it’s a matter of let’s get people foundational knowledge on these powerful topics and then help start them on their journey to get better with them.
Adrian Tennant: Great. You talk about the “data literacy umbrella” in your book. Could you explain what this means and how it relates to business decision-making?
Jordan Morrow: When we think about data and analytics in general, it is a big umbrella. There’s data strategy, there’s machine learning, there’s analytics, there’s data science, there’s coding, there’s statistics, data visualizations. That can be overwhelming. So, when we think about things from a data literacy perspective, where 90 to 99% of people do not need to go into each of those areas. I don’t want you to. The data space is massive. And if I was speaking at an event today in person, I might ask the question, “How many of you went to school for a background in data, like data and analytics, data science?” You’d be surprised how few people raised their hands. But what is interesting about that is how much money is being spent on these tools and technologies by organizations. So you’ve got this paradox occurring where, on the one hand, we’re spending a lot of money and expecting people to use it. On the other hand, we have a lot of people that don’t have skills to do it. There is a lot under that umbrella. So the number one thing I would tell people is you don’t need to dive into everything. You cannot boil the ocean very easily, right? It’s not easy. But what if you just take bite-sized models? So when you think about this entire umbrella that we’re looking at, think about it more specifically in relation to the four characteristics of data literacy. Maybe you don’t care about analyzing data right now because you’re not very comfortable reading it. Wonderful. Build a plan on how to read data better. Once you’re comfortable there, then it maybe becomes working with and analyzing it. And then that final piece is the communication. So the data literacy umbrella and data and analytics and now AI umbrella, they’re massive. And I think part of where we struggle is we take off more than we can chew right off the bat. versus a systematic step-by-step approach to say, this umbrella, which is supposed to keep us dry when it’s raining, well, I’m not going to hold it way over here to my right, and then the rain just falls on me. That doesn’t make sense. So I’m going to hold it above me, and I’m going to find the key critical elements that we’re looking at within that space and what area interests me the most. And then I can dive in, I can build a plan, I can study, and I can learn. That’s how we bite this off in an effective way to become better with it versus being overwhelmed by the massive amounts of things that are out there right now.
Adrian Tennant: Another interesting concept you discuss is the three C’s of data literacy. Can you explain what they are?
Jordan Morrow: With all the information at our fingertips, we probably have more information available to us – for good or bad, right? Misinformation, real information, truth, what people call truth, all this information sits out there. And the three C’s have probably never been more important than the history of this earth. And the three C’s are curiosity, creativity, and critical thinking. Let me start off with curiosity. For the majority of people, we’re not data and AI specialists. That’s not what they do. What I want them to do is get really curious about everything. Ask questions of everything. “Oh, this data point, what does it mean?” There’s a question. “Why does it say that?” There’s a question. For whatever reason, when we become adults, we get really bad at asking questions. We just take things at face value and we march forward. You know who are the greatest data literacy detectives on the planet? Children. I’ve got five of them. I want you to just observe kids one day. You know, you might be in the grocery store buying some food, shopping, you might have nieces and nephews, your own kids, and they are figuring things out regularly. Then we become adults and we stop. Not sure why. And in today’s day and age, with the amount of information at our fingertips, questions matter. Curiosity matters. Number two is creativity. Data and AI can be seen as boring, but maybe more importantly is we do not want to lose the creative human element from data and AI. Right now, you can see it. AI could create, I believe, very lifelike-looking people that you can’t tell is AI. It can write better than humans do. It can calculate faster than humans do. We cannot lose the human. AI and data need to be human-centric. One thing that humans have is creativity. And we may have forgotten that. Again, look at children. when they’re figuring things out, their imagination. We become adults, and no offense to a lot of us adults, we become dull, right? We become boring. Well, data and AI does not have to be boring. In fact, I want the human creativity to be a part of it in storytelling and dashboards and visualizations, the way we approach it, the questions we ask, be creative. Then number three might be my biggest sticking point with everything. With the amount of data at our fingertips, with the speed that we can receive that information, for whatever reason, we humans are really bad at critical thinking. We’re distracted. And that’s a very fair way of looking at it. Think about social media, how easy it is to be sucked in because social media knows what they’re doing, being sucked into our phones, being sucked into this and that, and not scheduling time to critically think about data and information. I encourage anyone who listens to this, block 30 minutes a week, you shut down all your technology, you write a problem on the board in a notebook and just start thinking about it, writing ideas down, potential ways to solve it for 30 minutes a day, and you are going to probably come up with more solutions in a week than if you didn’t block that off, possibly by a lot, because we’re critically thinking on it, right? Someone sends a tweet on X out. Do we just assume it’s right, or do we get curious, ask questions, we critically think on it? A news article pops up. Do we just think it’s right? Are we being curious and asking questions? Do we critically think? Far too often, we’re not. And those three key areas are essential for data and AI literacy, without a doubt.
Adrian Tennant: In your current role as SVP of data and AI transformation at AgileOne, how do you see organizations struggling with data literacy? What are some of the most common challenges?
Jordan Morrow: Oh, my goodness. So I started data literacy, the journey back in June 2016 is when I officially started to build the program. And I believe the world’s first full-scale program. And I had ideas, I think, before that. And the same issues you had back then are the same today. And it’s not just from a data literacy perspective, but it’s data analytics and AI in general. Number one, the full buy-in and investment of senior leaders is a challenge across the board. I think senior leaders know they need data. They know they need analytics. They need AI. The problem is, they don’t know what it takes to make it happen. You’ve got to be able to really help executive leaders understand why this matters, what it takes to get it done, and that it’ll take time. There’s no silver bullet. It’s not a quick fix. And that is not just data literacy. That’s across the board. Number two is the culture of the organization. The biggest roadblock you’re going to have to data and AI success is the people. You can have the cleanest data, the greatest data literacy program, and if people don’t want to use it, they’re not going to use it. Why would they? Even with mandates from above, they might use it a little more, but it’s still not going to get the take that you want. It’s really interesting that the majority of all of it boils down to the people. Now, obviously, you need good data quality, you need good tools and technology. I get all that. But again, the biggest challenge is the same thing we’ve been seeing for a long time is that the people aren’t absorbing it or adopting it, whether that is on the data teams or whatever. Now, there is an added element going on right now that is impeding it, too. That’s the speed of innovation with AI. That is operating at such a rate right now. Try keeping up. I live and breathe this stuff. I can’t keep up on everything. Now try executives who are just trying to stay afloat with it. So, this speed of what AI has done behind the scenes has caused another curveball into this scenario. All it still boils down to, though, is figuring out how data and AI help the business succeed. If you can do that, I don’t care the speed of AI, this or that, you’re moving hopefully in the right path. But that challenge in and of itself, this is why the same challenges that have existed for years are still here. A lot of it is the people and a lot of it is the communication.
Adrian Tennant: Let’s take a short break. We’ll be right back after this message.
![]() | Adrian Tennant: In partnership with our friends at Kogan Page, January’s Bigeye Book Club selection is “Be Data Literate: The Data Literacy Skills Everyone Needs To Succeed.” Author Jordan Morrow presents a practical guide for developing curiosity, creativity, and critical thinking to become data literate without requiring a data science degree. Through real-world examples and practical insights, “Be Data Literate” explains how to master analytics. As an IN CLEAR FOCUS listener, you can save 25 percent on “Be Data Literate” when you order directly from the publisher at KoganPage.com. Just enter the exclusive promo code BIGEYE25 at checkout. Shipping is always complimentary for customers in the US and the UK. “Be Data Literate” is your guide to thriving with data-driven marketing and advertising. |
Adrian Tennant: Welcome back.
I’m talking with Jordan Morrow, Senior Vice President of Data and AI Transformation at AgileOne, and the author of this month’s featured book, “Be Data Literate.” Jordan, in “Be Data Literate,” you outline a five-step plan for developing data literacy skills while partnering with AI. Could you share that with our listeners?
Jordan Morrow: Yeah, the five steps that I’ll share are kind of my mainstay. When it comes to developing, far too often we are focused on tools and technologies and skills. When it boils down to – Step number one is: “What am I trying to achieve?” That is your first step. Do not worry about what tools and technology you have. Do not worry what tools are available, what data is available. And that achievement could be twofold. From a business setting, it could be, “We are trying to achieve X, Y, Z from our business goals.” Great, we have our achievement, we have our outcome that we’re looking to achieve. On the personal front, it could be studying generative AI and how to prompt better. So we know what we’re trying to achieve, that is step one. Step two, we’re still not to the tools. Step two is: what is your strategy to achieve step one? How are you going to do it? Create a structured roadmap to get you there. It’s one thing to do a lot of talking around this stuff. It is better to take a lot of action, right? So we know what our objective is. Step number two is: set a strategy that’ll get you there. Step number three is: “Do we have the tools and technology to get us there?” Because if we don’t, why are we even going for it? Step four is: Do you have the right skills to get you there? And if not, build them. Then finally is Step five: Make sure your environment is right. Meaning it could be from a business perspective is the culture there. For me personally, I do a lot of journaling and reading in the morning before my whole family. Again, five children. Once that house gets rolling, it is not quiet and I need to be present and helping. But if I’m up before a lot of them, if not all of them, I can read in journals. So my environment is in the right position to help me succeed. So that environment, let’s go from the business setting. If you’re trying to drive a big data strategy, if your environment’s not ready for it, then stop. then restructure your five steps. And objective number one is getting your environment ready. Number two is the strategy that gets you there. Number three is the tools and technology to help support that. Number four is the skills. And then number five is the environment. Following that, you can use that to build data strategy. You can use that to do data literacy. You can use that to do AI, because it is a succinct five steps. And when you map that out, you’ll find I’ve got a gap in one of these, I need to reframe number one. That way it just becomes roadmap after roadmap of do this, accomplish this, do this, accomplish this. Again, not boiling the ocean, but a step at a time.
Adrian Tennant: You also write about the importance of being proactive rather than reactive with analytics. Jordan, could you explain what you mean by this and why it matters?
Jordan Morrow: Yeah, a lot of times, I think analytical work is, “Hey, we’ve got a fire we need to put out. Can you get me this data?” And I think that’s a lot of data and analytical work that exists. It’s we’re reacting to things. We are not guiding things. And that’s what we want to be thinking about with a proactive approach, meaning if all we’re ever doing is responding to questions, we’re probably not getting as far ahead as we should. Instead, we want to be proactive in our analytics. We want to set the tone in how we do data analysis, not have the tone set for us. We might have grand visions of things that we want to accomplish, but because others are the ones determining the route, we’re not getting as far ahead. And don’t get me wrong, you’re not going to get rid of reactive analytics. If you have a CEO or a senior leader saying, “I’ve got to answer this question by Monday, get to work,” you’re doing reactive analytics. But the better we get at roadmaps and structure and objectives and strategy, you might already have that answer for that senior leader before they even ask, because you’ve noticed it’s been asked five times over the last year. So, you build a proactive approach. By getting ahead of that game, you are the one setting the tone for your analytical world, building better predictions versus being stuck in descriptive analytics.
Adrian Tennant: Makes sense. For anyone who feels overwhelmed by data and knows they need to become more data literate, what advice would you give them?
Jordan Morrow: Step number one is I want you for a week to have a journal and just write how often you are using quote-unquote “data” to make decisions. Now, it might not be super advanced, but you already have data literacy. Did you fill up your tank with gas because you saw the gas gauge go down? “I filled up the car.” Those are data points. So number one, I want you to be more confident in your data skills than you realize you already are. Number two would be my three C’s. Be more curious, be creative, and critically think. Schedule 30 minutes a day to just think about things. And get really, really good at questions. Ask a lot of them. Be a good, healthy data skeptic. Become annoying to the data team. I’ve got five kids asking me questions all the time. How freaking annoyed do you think I get at times? Way too much. But they know me. I’m the guy who says, “Never stop asking questions,” even though I tell them, “Stop asking me questions!” But I want you to become that child again. Ask a lot of questions. “What does this data point mean?” “Why did you build it this way?” “Why’d you use that data visualization?” “How complicated is this?” “What’s prompting AI?” “What’s generative AI?” “What’s AGI?” I just came up with what? Six questions in a five-second span. Ask questions. And then the third thing is thinking about that umbrella and we’re gonna just make it smaller now. A data literacy umbrella with the four characteristics. Reading, working with, analyzing, communicating with data. One of those is the most important. Most of the time people say it’s communicating with data. That is incorrect. Because if you can’t read data well, you can’t work with it, analyze it, communicate it effectively. So if you don’t feel confident in reading data, get better at reading data. If you’re good at reading data, find other areas within those four characteristics to study and improve upon. If you need resources, you can connect with me on LinkedIn and I’ve got plenty I can share with you. I tell you what, though, Google or generative AI like ChatGPT are good ways to say, I’m trying to get better at working with data, I’m a beginner, what should I do? And just see what it says, and then move forward.
Adrian Tennant: If there was one key takeaway you’d want readers to get from “Be Data Literate,” what would it be?
Jordan Morrow: Oh, I keep coming back to the three C’s, but it’s probably those. And the reason is those three C’s can spur on a lot more data work, right? And if 90 to 99% of us are not data professionals by trade or title, your job might just be to ask good questions and turn it over to the data team who then receives those questions and does the dirty work. Number two is the creativity. Data and AI need to be human-centered. If you don’t think you’re creative, I call BS. You were a child once where you were probably creative, playing games, you know, doing something. Be creative. If it means improving a data visualization, it means getting creative with your stories or creative with questions. Be creative. Finally, I cannot emphasize this more and more and more. Critically think on the data and information being presented. Don’t take things at face value. I don’t care if it’s your CEO presenting the data. Ask questions. I’ve asked a question like that before. “How many of you would be comfortable questioning your CEO with their data?” Not as many people raise their hands. The culture needs to be such that everyone can be put on the spot and questioned on the data. If you know something is off, you better raise your hand, or then it’s on your shoulders that you didn’t. So, critically think on all the information. I know we keep talking about the three C’s, so let me add one other thing in there. Not everyone needs to be a data scientist. So, when you’re thinking about data literacy, AI, and all these things, you don’t have to be technical. You don’t have to be advanced. In fact, AI is making it so we don’t need as many technical people. That being said, though, I need everybody confident and comfortable in using it. And that’s what data and AI literacy should do.
Adrian Tennant: Great insights. Thank you. Jordan, if listeners would like to learn more about your work or your book, “Be Data Literate,” what’s the best way to do so?
Jordan Morrow: Number one thing is following me on LinkedIn. I connect, I mentor, I post quite regularly. It’s just to follow me on LinkedIn. You can see how I speak about things. You’re probably getting a good vibe from this podcast on how I discuss it. But number one would be follow and connect with me on LinkedIn. Number two, you could go right to my publisher, Kogan Page Publishing. They’re wonderful. They’ve published my four books and I’m in line right now to write book five. It’s been approved. So a wonderful publisher. If you go to Kogan Page Publishing and search for Jordan Morrow, you could get all four books pulled up right there.
Adrian Tennant: Perfect. And you’ve just got, I believe, the fourth book being published now. Do you want to tell us about that?
Jordan Morrow: It just barely published. In fact, I’ve got to give a thank you to my publisher. So given that my publisher is in the UK, I say they publish internationally first, but for them it’s not international because they’re in the UK. It’s always everybody but the US and Canada. And so December 3rd is when it launched internationally. And then I got a message on December 31st from someone at my publisher saying, “Congrats.” I’m like, “Oh, I forgot it’s today.” So a huge shout out to them reminding me that on New Year’s Eve, I think it was, here in the US and Canada, my fourth book launched, which is “Business 101 for the Data Professional.” So it’s somewhat of a flip of “Be Data Literate.” “Be Data Literate” is for non-data professionals. And then flip it, I basically built a business literacy for the data professional. That being said, both books are good to have in a library. The way I approach it is, again, not from an academic, here’s a four-year degree that you had to sit through, but hopefully, principles that empower people to be much more successful in these spheres that are now just everywhere.
Adrian Tennant: Jordan, thank you very much for being our guest this week on IN CLEAR FOCUS.
Jordan Morrow: Thank you so much for having me.
Adrian Tennant: Thanks again to my guest this week, Jordan Morrow, the author of “Be Data Literate.” As always, you’ll find a complete transcript of our conversation with timestamps and links to the resources we discussed on the IN CLEAR FOCUS page at bigeyeagency.com. Just select ‘Insights’ from the menu. Thank you for listening to IN CLEAR FOCUS, produced by Bigeye. I’ve been your host, Adrian Tennant. Until next week, goodbye.
TIMESTAMPS
00:00: Introduction to AI and Data Literacy
00:19: Welcome to IN CLEAR FOCUS 18th Season
00:40: The Importance of Data in Decision-Making
01:11: Defining Data Literacy
02:38: Understanding Data Literacy
04:19: Four Levels of Analytics
07:38: Updating “Be Data Literate”
09:29: The Data Literacy Umbrella
11:49: The Three C’s of Data Literacy
15:08: Challenges in Data Literacy
17:46: Break and Book Promotion
18:51: Five Steps to Develop Data Literacy Skills
21:53: Proactive vs. Reactive Analytics
23:20: Advice for Overcoming Data Overwhelm
25:28: Key Takeaways from Be Data Literate
27:16: Connecting with Jordan Morrow
27:53: Introduction to Jordan’s Fourth Book
29:02: Closing Remarks