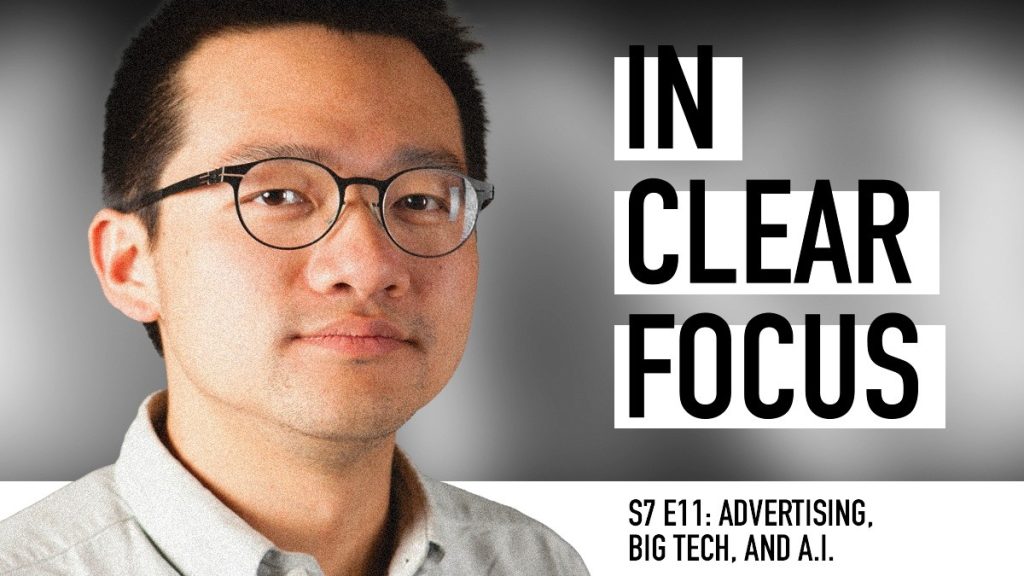
Dubbed “the busiest man on the internet”, Tim Hwang joins us to discuss his book, Subprime Attention Crisis. Tim explains how and why he believes the programmatic display advertising ecosystem resembles financial markets prior to the subprime mortgage crash of 2008. We talk about security vulnerabilities when sharing ad data, geofencing, and Washington’s increasing scrutiny of Big Tech. Tim also provides his unique insights into the uses and abuses of artificial intelligence in advertising.
Episode Transcript
Adrian Tennant: Coming up in this episode of IN CLEAR FOCUS:
Tim Hwang: There are a number of similarities between the kinds of markets that we see in advertising today and the kind of practices that existed during the 2008 crash, and not just the 2008 crash, but sort of market bubbles in general.
Adrian Tennant: You’re listening to IN CLEAR FOCUS: fresh perspectives on the business of advertising produced weekly by Bigeye. Hello, I’m your host, Adrian Tennant, VP of Insights at Bigeye. An audience-focused, creative-driven, full-service advertising agency, we’re based in Orlando, Florida, but serve clients across the United States and beyond. Thank you for joining us. Today, we’re living in a global economy with virtually unlimited access to the world’s information thanks to the technology and connectivity offered by the internet. Because their business models are based on advertising, many of the largest tech companies offer digital services to consumers at no cost. Certainly, we’ve come a long way since the appearance of the first-ever banner ad for AT&T, which appeared on Hotwired.com back in 1994. In his 2016 book, The Attention Merchants, Columbia law professor Tim Wu characterizes consumer attention as the defining industry of our time. Digital advertising is omnipresent today thanks to mobile devices that are always on and rarely leave our sides. To illustrate the point, Google’s Chief Marketing Officer, Lorraine Twohill, recently stated that an individual sees almost 2 million ads per year. That’s well over 5,000 each day. But the attention economy is coming under increasing scrutiny from within the ranks of the advertising industry itself and from federal agencies. Once dubbed “the busiest man on the internet” by Forbes, Tim Hwang is a writer, lawyer, and technology policy researcher. Previously, Tim was Google’s global public policy lead on artificial intelligence and then director of Harvard University’s Ethics and Governance of AI Initiative. Today, Tim is a research fellow at the Center for Security and Emerging Technology at Georgetown University. Tim’s book, Subprime Attention Crisis: Advertising and the Time Bomb at the Heart of the Internet, was published last year and investigates the ways in which big tech monetizes user’s attention. Tim suggests that the internet has a precarious future, likening the programmatic ad ecosystem to the housing bubble of 2008. To talk more about this, as well as artificial intelligence and Big Tech, Tim is joining us today from his home office in New York City. Tim, welcome to IN CLEAR FOCUS!
Tim Hwang: Yeah, thanks for having me on the show, Adrian.
Adrian Tennant: The central idea explored in your book is that the digital programmatic advertising ecosystem is at risk of collapsing. Before we dive into your thesis, what was the experience or insight that led you to write subprime attention crisis?
Tim Hwang: Well, it’s funny, actually, Adrian, as you mentioned, I spent a few years at Google, where I was director of global public policy for AI and machine learning. And one of the interesting things that I observed when I worked at the company was – obviously Google is one of the companies that is at the heart of this debate about advertising online and a huge amount of its revenue comes from programmatic advertising – but what’s really interesting on the day-to-day basis is that Google, you know, the culture of the company, doesn’t actually have people talking about ads all the time. People are interested in self-driving cars and artificial intelligence. And what’s amazing is even when you talk to engineers at the company, you ask them, “So how do we make money?” And people will say, “Oh, advertising of course.” And then you say, “But really, how does it happen?” And it turns out that most people, even people at these tech companies, just have a kind of vague sense of how these companies actually make money. And so, you know, the origin of the book was really just attempting to understand this ecosystem and to kind of create an accessible way for people to really get into the guts of this infrastructure that is responsible for some of the biggest companies in the world. And that’s really kind of the spark that led to the book.
Adrian Tennant: Well, in the book, you highlight the fact that the commodification of the internet has enabled significant economic growth, as well as the development and provision of online services that many of us consider utilities at this point. But you worry about the extent to which many services we take for granted are at risk if advertising revenues were to collapse. So Tim, how did we get to this point?
Tim Hwang: Yeah. You know, one of the interesting things about the history of the internet is that I would say nowadays we take for granted that so many platforms and services on the internet are monetized through ads. But this actually really was not the assumption when the internet got started. It’s very funny. If you look at the original business model for Google, they assumed that actually a small, tiny amount of their revenue would come from ads and that they would actually make money licensing their algorithm to companies, which is such a quaint business model in some ways. And, what we discovered and have discovered over the last few decades is just that advertising has turned out to be such a prolific and powerful way of generating huge amounts of money extremely, extremely quickly. Because essentially, what you’re able to do is offer a service for free, which makes it really attractive to people. They don’t have to pay anything to get the benefit from these services. And, on the other hand, basically, because this company can grow so quickly, they can simultaneously monetize through ads. And so it maximizes growth from a user standpoint but also maximizes growth from a revenue standpoint. And that has just turned out to be a very attractive model, almost to the extent where nowadays the assumption is that you will do an advertising-driven business model. And if you’re trying to do an alternative, that’s kind of the burden that you have to overcome, right? Investors will look at you and say, “You know, is this subscription business model really going to work? Why aren’t you doing advertising?” And I think that’s mainly the reason that we ended up where we are.
Adrian Tennant: The enabling technology of programmatic advertising is real-time bidding or RTB for short. Tim, how does RTB resemble the financial trading practices that led to the 2008 crash?
Tim Hwang: So I’ve observed this to a few people, you know, it’s very funny. I was talking to a friend who is like, “Oh, you know, usually when people deploy metaphors in a book, the idea is to try to take a complex system and make it more understandable.” But the irony here is basically that the whole core of the book uses a thing that is famously complex. If you read the book, you’ll have to be the judge as to whether or not this is a successful strategy at all. But I do think the core of the book is really to argue that there are a number of similarities between the kinds of markets that we see in advertising today and the kind of practices that existed during the 2008 crash and not just the 2008 crash, but sort of market bubbles in general. And I think that’s a good way of thinking about it without having to get too far into the weeds. Which is basically that there’s always a couple of ingredients that we see throughout history that are really associated with a market bubble. You know, the three components my argument would be is: Opacity – it’s very difficult to see what’s going on in a marketplace. Perverse incentives – you have people who have a lot of incentives to boost the perceived value of a market without really, having to recognize reality. And then finally you have declining asset value – basically, the thing being traded that we think is so valuable is getting less and less valuable over time. And so what you end up with is a market where you can’t really see what’s going on. There are lots of people telling you that everything is great, but the thing that’s actually being sold is not so great. And that creates the bubble, right? Because when people realize that, things are maybe not as great as they seem, there’s panic in the marketplace. And the claim is basically that what’s happening in programmatic advertising has a lot of similarities to the three characteristics that I just mentioned. So one of them is opacity. It actually turns out that it’s really, really difficult to see what’s going on in the marketplace. There’s a huge amount of, fraud. There’s a huge amount of inaccurate metrics. there’s a huge amount of brand safety issues, and these are all really difficult for people to figure out in part because we’ve generated such a massive system that’s just difficult to monitor. We secondly have a group of people who have very perverse interests, one of the stats is that about 50 percent, if not a little bit more than that of every dollar spent on programmatic advertising is consumed by an ad tech company. Well, that tends to create a huge incentive on the part of the ad tech company to keep this casino rolling whether or not it’s actually really effective for their clients. And then finally we have declining asset value, which is that there’s been an assumption for a very long time that programmatic advertising works on a fundamental level, that you can direct a message to a person and get them to do what you want them to do either to vote for a candidate or buy a product. And I think there’s increasing evidence that that’s actually not the case. And so when you add these three things together, at least for me, what I kind of squint and turned my head, and I say that starts to look like a market bubble, not just from 2008, but just the whole history of market bubbles kind of suggest that this is same dynamics at work.
Adrian Tennant: In spite of the economic uncertainty during the COVID-19 pandemic, digital ad revenues actually continued to climb. US programmatic digital display ad spending grew 10 percent in 2020 to $66 billion. This year, eMarketer predicts revenues will reach $81 billion. Tim, what do you think could trigger a collapse in programmatic advertising as severe as the 2008 subprime mortgage crisis?
Tim Hwang: Sure. And I think this is actually where I think a comparison to things like 2008 is actually very helpful because just because a lot of money is flowing into a market, it’s a fallacy to believe that that tells us that the market is healthy. I think one of the worst arguments I’ve gotten from people in the advertising industry who hate the book says, “Well, how could all these billions of dollars be wrong?” And I say: look at the 2008 crisis. On the eve of the financial crisis of 2008, people basically said, “Well, look, there’s so much money flowing and how could it possibly be going wrong?” And so I don’t think that’s an indicative fact. All that tells us that there are people spending money – it doesn’t necessarily tell us whether or not the market is healthy or not. Now, I think you ask the $81 billion question, which is “Okay, what could cause the collapse?” And I think one of the things that I’m looking at very closely, is actually what’s happening in the regulatory space. So what we see right now is privacy laws being passed in Europe. California has a law called the CCPA. There’s a bunch of state-level laws in the US that are doing the same. And, you know, the advertising industry has made the argument that you’d expect them to make, which is “Don’t put these regulations into place because it will kill our business. If we don’t have this data to target, then we can’t possibly operate as a business.” I actually think that there might be a very perverse outcome from these laws where the laws go into place, advertisers lose access to the data, but it turns out the advertising is okay. That it actually doesn’t really change the effectiveness of ads. And I think that actually triggers a potential collapse because everybody suddenly says, “Okay, so what have we been doing all these years, collecting all of this huge amount of data about people? What does all this behavioral targeting do? What is all this artificial intelligence to do in targeting ads?” And if it all turns out that that was worthless in the end, that we ran this experiment, it turns out that you don’t really need access to this data. I think that is the kind of a shock to the system that could really cause a collapse.
Adrian Tennant: Digiday published a story about Democratic Senator Ron Wyden’s proposed legislation that could place restrictions on ad-tech data flows outside the US. Magnite and Twitter, among others, have ad tech partners based in countries deemed high risk. In a statement sent to Digiday, Senator Wyden says “There’s a clear national security risk whenever Americans’ private data is sent to high-risk countries like China and Russia, which can use it for online tracking as well as to target hacking and disinformation campaigns.” Tim, do you agree with the senator’s position?
Tim Hwang: I agree and disagree is actually how I’d address this question. Because I do think that my argument kind of cuts in a couple of different ways. And one of the unexpected ways that you might be interested in, how this argument cuts is that basically, it cast some doubt on whether or not disinformation campaigns – micro-targeting – whether or not that all actually works. I thought one of the most interesting things coming out of the Cambridge Analytica scandal was that the British privacy regulators did a post-mortem with an agency called the ICO. And that agency concluded that even though there was a huge privacy violation in the Brexit vote, it turned out that they couldn’t find any evidence that any of that psychographic targeting did anything at all in terms of actually influencing the vote. And so I guess I disagree with Senator Wyden when he says that the risk here is that it facilitates disinformation or influence campaigns. I think we should be rightly skeptical as to whether or not all of this data actually amounts to anything. Now, on the other hand, though, I think that the correct argument is, well, we should still take action because it’s a huge privacy violation. And I definitely agree with Senator Wyden there. There was a great paper that came out of the University of Washington a few years ago that concluded that you can use geo-fencing in the advertising system to basically monitor when an individual person is at their house or going to work or whatever. And a lot of our research suggests that there’s a lot of these data leakages in targeting ads that really are quite privacy-invasive. And I think it actually in some cases of national security risks because it might be really useful to know when are people going to the DOD? When is this person moving from one military base to the other? You can actually turn that you can facilitate some of that through the advertising ecosystem. It’s an unintentional side effect, but I think a real national security risk.
Adrian Tennant: Well, concern about big tech has emerged as a bipartisan issue. This year has seen the publication of both Antitrust authored by Democratic Senator Amy Klobuchar and The Tyranny of Big Tech by Republican Senator Josh Hawley. How do you see things playing out for big tech companies over the next two to three years, especially with Lina Khan, who’s been critical of big tech, now heading up the federal trade commission?
Tim Hwang: Yeah, it is actually really interesting how it’s evolving in DC right now because, needless to say, we don’t live in a particularly bipartisan time – probably understatement of the century! But it is interesting to me that whether you are a hardcore progressive or a diehard Trumpist it actually has turned out that both sides agree that something needs to be done about Big Tech. And so I do think that the next two or three years really are the Super Bowl, if you will, of tech policy. That if something big is going to happen, it’s going to happen in the next few years when there’s clearly big public concern and big policy concern about these companies and what they’ve become. Now there’s actually an interesting question about the Lina Khan and her view around some of these things. And particularly relevant to your question, because just today, Amazon actually filed a petition trying to get Lina Khan disqualified from participation in antitrust prosecutions, arguing that basically she’s prejudged the situation given her previous publications. And so I think the fight is really becoming a knife fight, and I think it actually is getting quite dirty in some ways, but I do believe that I think the tech companies are on defense now. And I think really now the question is, can we really articulate what we think is the right way forwards? Like what is the kind of internet ecosystem that we want to live in? And I think this is where maybe the bipartisan consensus breaks down. I think this is the trouble is can we move beyond simply having hearings where we yell at Mark Zuckerberg to actually making real policy in this space? That is the challenge that I think we find ourselves faced with, but I think if there was a time for it to happen, it’s going to happen in the next few years.
Adrian Tennant: Let’s take a short break. We’ll be right back after this message.
Sandra Marshall: I’m Sandra Marshall, VP of Client Services at Bigeye. Every week, IN CLEAR FOCUS addresses topics that impact our work as advertising professionals. At Bigeye, we always put audiences first. For every engagement, we’re committed not just to understanding our clients’ business challenges but also learning about their prospects and customers’ attitudes, behaviors, and motivations. These insights inform our strategy and collectively inspire the account, creative, media, and analytics teams working on our clients’ projects. If you’d like to put Bigeye’s audience-focused consumer insights to work for your brand, please contact us. Email info@bigeyeagency.com. Bigeye. Reaching the Right People, in the Right Place, at the Right Time.
Adrian Tennant: Welcome back. I’m talking with Tim Hwang, a research fellow at the Center for Security and Emerging Technology at Georgetown University. Tim, let’s talk about artificial intelligence. We’re seeing AI and machine learning in advertising and marketing contexts more and more often. Cognitiv – spelled without an ‘E’ – describes itself as the leading custom AI delivering adaptive algorithmic advertising. It promises to – quote – “predict consumer behavior and drive full-funnel marketing performance at scale through the power of custom deep learning solutions” – end quote. Our podcast guest last week was Melanie Deziel, the first director of branded content for the New York Times and an expert in content strategy. We talked a bit about some of the AI-assisted writing tools now available, many of them based on technology created by OpenAI called Generative Pre-Trained Transformer 3, or just GPT three for short. Tim, could you give us some background on this technology and why we’re seeing new tools now?
Tim Hwang: Sure, I can definitely do that. I’ll give you the 30-second explanation of machine learning and so you can, bring that hopefully into your work if you’re listening to this podcast. One way of thinking about how we’ve programmed computers in the past has been that we basically program explicit rules into a computer. So imagine a task where we say, “Okay, we want to teach a computer basically how to recognize a cat in a photo.” Well, the old way of doing it basically is that we get a bunch of smart people together. They would stroke their chins and say, “Okay, well, cats have pointy ears, and they’re fluffy, and they fall within these kinds of colors. So let’s program those rules into a computer. And when a computer can see a photo, they can say, “Oh, does it have pointy ears? Does it have these colors? Is it fluffy?” And really for a long time, one of the sort of big ideas in computer science has been the notion of doing machine learning, which is okay rather than us explicitly programming rules into the computer, how about we just show the computer a bunch of photos of cats and have it guess basically. We say “Computer, is this a cat or a dog?” And he’ll say, “Oh, I think that’s a dog.” And you’ll say, “Oh no, you got that wrong. That’s actually a cat.” And it turns out if you do this with millions of images, sometimes even billions of images, the machine actually gets really, really good at figuring out how to do this without us having to explicitly program in rules. And it can do this basic cause it’s really good at identifying patterns in data. Now for a really long time, the reason this was considered a big dead end was in part because we were missing two big things. One of them is that we just didn’t have a whole lot of data lying around. So when they were working on the set in the fifties, you would say, “Oh, I have 20 Polaroids of cats” and it turns out the machine can’t just learn with that small amount of data. The second one is that the kind of computational tasks that need to happen in this process are pretty computationally intensive. And it turns out that in the 2000s, we sort of figured out what was the right kind of hardware infrastructure, the right sort of chips to really make this happen in a really powerful, efficient way. And so, because we have these two things, we’re actually finding that machine learning is really, really good at a number of tasks. So for image recognition, it actually turns out to be a great technology. Now, some of the examples that you quoted are in the advertising space and I think the dream of AI in advertising is the notion of “Okay. You know, we have all of this data about consumers, could a machine do a better job at figuring out what they want and what kind of messages will be credible to them that a human might not be able to do?” I tend to be a little bit of a skeptic here. Some of the things that we’re finding is that ironically, AI algorithms in advertising find people who would have bought the product anyways. That’s in fact how good they are – is that they have patterns about who bought your products and they just find more of those people. And so one of the big questions is whether or not these AI solutions in ads really end up shifting people’s behavior, or if it’s really kind of correlation rather than causation. “We show you an ad, but you would have bought the product anyways.” so I think we’re still working through some of these things, but that’s kind of the quick nutshell of the technology, why we’re seeing it, and I think, I guess my view on its application, in the ad space.
Adrian Tennant: Well, I watched a webinar a few days ago in which an influencer demonstrated her process for creating three months’ worth of social posts in just three hours using a GPT-3 writing tool. Tim, as a writer yourself, how did do you feel about an internet filled with AI-generated content?
Tim Hwang: Yeah. And you’re actually touching on a nice little kind of Coda to the explanation that I just gave around AI. As I mentioned, really what you’re doing in machine learning is you’re trying to get the machine to understand a pattern, right? Whether or not that’s a pattern of how cats look or the pattern of the way a stock moves up and down. And one of the interesting things that we’ve discovered is once a machine learns those patterns, you can actually configure it so it spits out more examples of what it’s learned in ways that have never existed. So you basically trained it on a number of images of cats. It can generate infinite images of cats that have never existed before. And this is what we’re seeing with technologies like GPT-3, which is basically you train a machine learning algorithm on all the text on the internet, and actually turns out that it ends up being pretty good at writing articles. And so I personally am really excited by experiments in this space, that I do think that one of the really interesting things we’re going to find out is what are the kinds of writing that is really easy for computers to do, and what is the kind of writing that makes it really, really hard? And then additionally, I think there’s a social question, which is what are we comfortable with machines writing? And at least for me, I have a friend, Robin Sloan, who’s a science fiction writer, who does a lot of these experiments in kind of programmatic writing. And from some of the things I see, I do really believe that we might go into a future of sort of computer-assisted writing, which actually could be really exciting. And actually produce more creative work than a human writer working alone. And I, that doesn’t scare me, I think that’s actually something that’s worth experimenting and exploring.
Adrian Tennant: So you would see these as assistive tools rather than authors in their own right?.
Tim Hwang: I mean, let’s see if they can be authors in their own right. I mean, I do think that, you know, my long bet is in 10 years, we will have a totally programmatically-generated book that hits number one, that actually happens.
Adrian Tennant: Well, McKinsey just published a report identifying 56 foundational skills that they believe citizens will require in order to future-proof themselves for the world of work, defined as distinct elements of talent or DELTAs. Among those with the highest correlation to a person’s level of education are digital literacy, programming, data analysis and statistics, motivating different personalities, and interestingly, inspiring trust. Broadly, what kinds of issues should governmental agencies be thinking about in terms of the relationships between human capital and AI systems?
Tim Hwang: Yeah. So this is a deep question and something that I think a lot about. And it is interesting. Before I kind of launch into my answer, I will sound a note of disagreement with the McKinsey report. Actually, I think one of the interesting things that you should maybe avoid working in is actually programming. One of the interesting things that we’re finding actually is that AI systems are actually pretty prolific at generating code. And we might actually live in a world where a lot of the work that developers do on a day-to-day basis gets replaced by machines. And so you have computers programming themselves, which I think is like a fascinating outcome but it’s also, I think, something that we need to think seriously about. Which is, is this thing that we’ve seen, or thought of as like, “Oh, you really need to go into computer programming. That’s really the growth industry.” You know, in some ways, by the time we are thinking that it’s already too late. People are finding ways of commoditizing it and automating it. And it actually may not be the skill that you really want to invest in in the future. Now, I think one of the big questions actually, you know, to go to your broader point about what should government agencies be thinking about in terms of the relationship between human capital and AI systems? Is that we frequently kind of think about AI systems as just having defined effects in society, right? So people say, “Oh, you launch an AI system and jobs get replaced. That’s just the way it is.” But I do think that we, as a society, have the ability to shape how our systems are designed and how they are implemented in society. There are actually very deep reasons why society says, “Oh, well, either we could have a robot assist a warehouse worker” or, “Well, actually we just want it entirely replace the warehouse worker.” Those are actually political choices and those are policy choices that are being made. And so one of the things I think that sort of governments really need to be thinking about is not necessarily what will technology do to us, but more actually, how do we want to shape technology’s integration into society? And to realize that we actually have an ability to kind of shape what role we actually want tech to play. And I think that’s one of the biggest things that I would kind of urge government agencies thinking about these issues to kind of keep in mind as they craft policy and whatever it is, you know, self-driving cars, labor rights, you know, the regulation of capital markets, right? I think these are all kind of tied together.
Adrian Tennant: Computers writing code without human intervention? Tim, I’ve got to ask, you how close are we to the singularity?
Tim Hwang: So I am a deep singularity, skeptic. I feel like everything that we’ve seen in technology actually is incredibly powerful, but incredibly narrow systems. So for example, the Deep Mind system that beat the Go champion a number of years back – that system’s never going to wake up one day and decide what it really wants to do is drive cars. And so what we do is we have basically is ultimately in many cases, we still set the parameters of the technology and what we want it to do. And so I am very skeptical about the idea of these systems escalating out of our control. More typically, what will happen is that the sort of incompetent deployment of these technologies or the overly optimistic or naive deployment of these technologies is really where most of the harm is going to be versus a Skynet type situation.
Adrian Tennant: Tim, how do you foresee artificial intelligence evolving over the next few years?
Tim Hwang: Yeah. So some of the most interesting things I think that are happening in AI, really, I think there’s two trends that I’ll point out. One of them is I think we’re moving from a phase in which AI was very much kind of like a lab experiment – like something that nerds put together and demonstrated that he can do incredible things with – to the realm of sitting back and being like, “Okay, so what is it actually good for, and how do we actually make it usable by people who don’t have a PhD in machine learning?” And so I think there’s a bunch of really interesting work that’s going to happen over the next few years, looking and thinking about what is the UI and the UX of AI, how do we interact with these systems? How do we make them usable? How do they signal to us that something is going wrong? These are actually really deep issues that I do think that there’s a lot of really interesting work happening. A second domain that I think is really interesting is that, one of the things that we’ve learned from these technologies is that if you deploy them incorrectly, a lot of bad things can happen. And one of the things that can happen is, AI systems are kind of dumb in their way, right? Like they basically just learn the patterns in the data that we give them. And so there’s been really interesting incidents where we say, “Okay, we train a facial recognition algorithm on only faces that have lighter skin tones.” And it turns out that actually then people are not sort of recognized if they have darker skin tones by the system. And so I do think that there is a whole lot of research work and also in fact, frankly, kind of political work to be done thinking about okay, so how does society really want to create requirements around these systems, right? We’re going to deploy a facial recognition system – A, do we want it at all? And then B, if we do right, like how should it be designed? What are the requirements for it to be designed and deployed? And I think that kind of universe of fairness in machine learning, but I think obviously it’s a much broader concept than that, is going to be where a lot of the action is over the next few years..
Adrian Tennant: Let’s switch gears. Tim, back in 2009, you created The Awesome Foundation, which has a stated mission of “forwarding the interest of awesome in the universe, $1,000 at a time.” Tim, can you tell us what the Foundation is and how it awards grants?
Tim Hwang: Sure. So the origin of this project, basically I had a couple of friends who, were applying to foundation grants, you know, they were trying to like get money for their art projects or their research projects or what have you. And all of them would go into this with a whole lot of enthusiasm and basically come back a little later incredibly depressed. And the reason they were incredibly depressed is because if you’ve ever applied for a grant before, you know the process is extremely bureaucratic, extremely slow, and, really raises the question as to whether or not it’s worth the money at all. And so we kind of started The Awesome Foundation as in some ways like a punk rock philanthropy, if you will. Really simple idea: you get 10 people together. They each contribute a hundred dollars a month and it creates a thousand-dollar grant. And that grant is basically just given no strings attached in cash. Sometimes we just give it in a paper bag to someone, To do a project that is awesome. And there’s no other criteria than that. And it turns out when you do that, there’s actually a lot of projects that are just waiting to be done, that are kind of unleashed by this way of giving out grants. And so a number of years later, we’ve given out a few million dollars, there’s chapters all around the world, and it’s really become kind of a network of giving circles around the world that give grants to sort of weird sort of oddball type things, projects. And that really is the case and a core of The Awesome Foundation is a sort of community of people I would say, that are committed to giving these fun grants, often in their local neighborhood or city.
Adrian Tennant: Do you have some favorite examples of projects that have been funded?.
Tim Hwang: Yeah. Well I think one of my favorites, so the chapter in DC is a very active chapter. They’re really awesome community. And they funded someone a number of years back, who basically converted an alleyway in their neighborhood to a simulation of the Indiana Jones Temple of Doom, with big boulders rolling after you as you run away as part of the experience. That you’d be able to go in, you would get to put on a leather jacket and like a hat and they would push this big inflated boulder towards you as you ran away while, of course, the music played. And it was just so great. It was like a very fun, cheap project. That was kind of a fun thing to have in the neighborhood. And I think really kind of captures in some ways, the sort of like spirit of the type of projects that happen through the Foundation.
Adrian Tennant: If IN CLEAR FOCUS listeners would like to learn more about you, your work at the Center for Security and Emerging Technology, your book, Subprime Attention Crisis, or get involved with The Awesome Foundation, where can they find you?
Tim Hwang: Sure. Yeah. So I’m just @TimHwang. So Tim Hwang.org. The .com, I believe, goes to a Korean pop star of the same name, which is not me. And I’m also on Twitter, on the same name. And that’s probably the best way to keep up with my projects.
Adrian Tennant: Tim, thank you very much for being our guest this week on IN CLEAR FOCUS.
Tim Hwang: Yeah. Adrian, thanks for having me.
Adrian Tennant: Thanks to my guest, Tim Hwang, author of Subprime Attention Crisis and a research fellow at the Center for Security and Emerging Technology at Georgetown University. You’ll find a transcript with links to the resources we discussed today on the IN CLEAR FOCUS page, at bigeyeagency.com under “Insights.” Just click on the button marked “Podcast.” If you enjoyed this episode, please consider following us on Apple Podcasts, Spotify, Google Podcasts, Amazon Music, Audible, YouTube, or wherever you listen to podcasts. Thank you for listening to IN CLEAR FOCUS produced by Bigeye. I’ve been your host, Adrian Tennant. Until next week, goodbye.