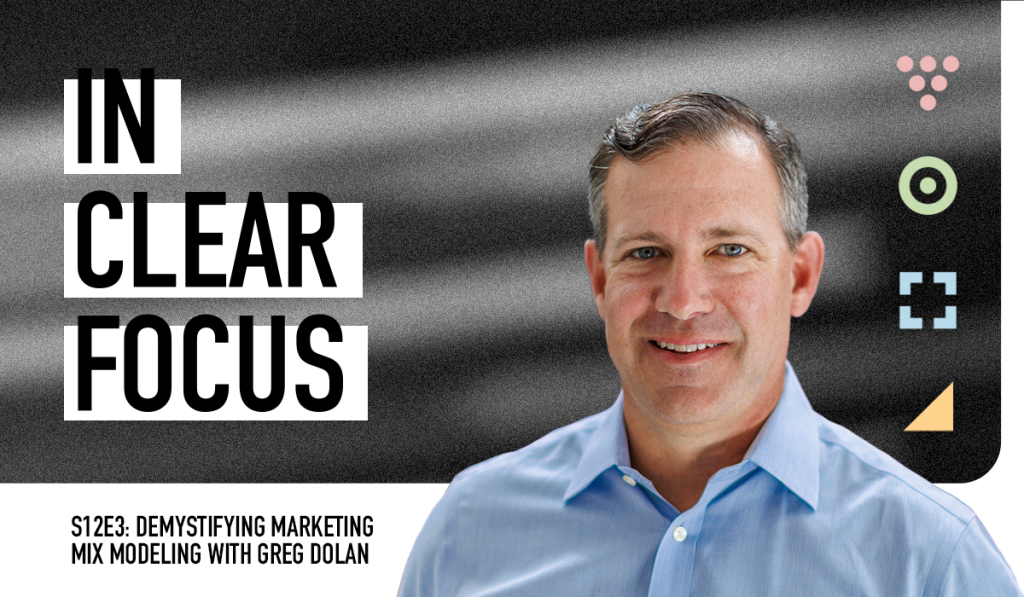
Greg Dolan of Keen Decision Systems joins us to demystify Marketing Mix Modeling. Greg explains how Keen’s AI-driven model offers real-time insights and simulations, facilitating financial optimization and long-term brand value creation. Greg emphasizes the need for continuity in marketing, citing case studies that illustrate how going dark can result in loss of profitable demand. Learn how MMM supports decision-making with Keen’s predictive and prescriptive planning outputs.
Episode Transcript
Adrian Tennant: Coming up in this episode of IN CLEAR FOCUS.
Greg Dolan: There’s a big difference between what was traditionally marketing mix consulting and what we do. The use case for both marketing teams and agency partners is that they’re using this as a planning tool that they can understand and simulate outcomes and then execute those plans and be able to validate the original plans through the system.
Adrian Tennant: You are listening to IN CLEAR FOCUS, fresh perspectives on the business of advertising produced weekly by Bigeye, a strategy-led full-service creative agency growing brands for clients globally. Hello, I’m your host, Adrian Tennant, Chief Strategy Officer. Thank you for joining us. Today’s media environment is increasingly complex, with a myriad of online and offline channels available to marketers. Understanding hybrid consumer journeys and the effects of advertising in newer channels like retail media is a significant challenge. Economic uncertainty, tighter privacy regulations, and the death of the cookie have all renewed interest in one analytical tool, in particular: marketing mix modeling, or MMM for short. It promises to provide marketers with a holistic approach to planning and media optimization by combining data from multiple sources to generate historical analysis and future marketing planning scenarios. To discuss marketing mix modeling, we’re joined today by Greg Dolan, the co-founder, and CEO of Keen Decision Systems, an Inc. 5,000 software-as-a-service company whose unified measurement and optimization platform enables brand marketers to make data-driven decisions. Prior to co-founding Keen, Greg had brand and corporate strategy experience at CPG companies, including Kraft Foods, Campbell Soup Company, Nabisco, and Mondelēz. He frequently contributes to research publications and is a guest lecturer at UNC Keenan Flagler Business School, teaching MBA students advanced analytics. To discuss how marketing mix modeling can help brands understand the effectiveness and efficiency of their marketing investments, Greg is joining us today from Keen Decision Systems’ offices in Durham, North Carolina. Greg, welcome to IN CLEAR FOCUS!
Greg Dolan: Thanks, Adrian. It’s great to be with you.
Adrian Tennant: I gave a brief description in the intro, but can you tell us more about your background before co-founding Keen Decision Systems?
Greg Dolan: Absolutely. My background really led to the creation of Keen, so I started my career as a consumer packaged goods brand marketer. So I worked in the food industry at Nabisco and then Kraft Foods and the Campbell Soup Company, and I was primarily in general management-focused P&L management of brands. I had responsibility for large marketing budgets. And my biggest lever in generating profitable demand was marketing. And unfortunately, I had, you know, old fashioned marketing mix, where I had a marketing mix study that was delivered, you know, once a year, about six months after the data was delivered to the consultant. It gave me a report card of what happened in the previous two years, but it didn’t give me a good understanding of what I do next. And as a P&L owner, as someone managing a business, I really had to understand, you know, “How do I make better decisions based on the information I have?” So I was lucky enough to meet my technical co-founder, John Busbice, who had been doing marketing mix analytics for the pharmaceutical industry, and he was tired of putting together the marketing mix presentation, a 200-page PowerPoint deck, and delivering it to marketers for it not to be used for decision making. So we felt together there was an opportunity to disrupt the market, develop a next-generation solution that was delivered through software, and not only really focused on just measurement, but more importantly, started to guide decision-making as information became available, and really tie that directly to the financial outcomes that the brand was being held accountable for.
Adrian Tennant: Well, in the context of today’s still uncertain economic outlook, an article you wrote for Quirks recently discussed the shortsightedness of companies slashing their marketing and advertising spending. Greg, why is it important to maintain long-term branding efforts regardless of the prevailing economic conditions?
Greg Dolan: Well, as marketers, we know that marketing drives value and value over time. And I think that this is missed in a lot of traditional marketing analytics. So what we’ve been able to do as a system and as a software application is account for the timing impact of marketing. So in our system, we were able to build in long-term effects and really understand how each dollar of marketing is impacting both short-term revenue and long-term value. And what we found was – and this is supported by Ehrenberg-Bass and others who really, you know, focus on continuity of marketing – is that when you cut marketing, and you go dark and even flight the business in intervals, then you’re not in front of the consumer. And that marketing that you have put into the marketplace and deployed starts to decay, and it takes that much more spending and time to get the same amount of volume back. So it’s more expensive over time to build back after you’ve gone dark and cut budgets and not invested in the consumer.
Adrian Tennant: I probably gave an overly simplistic description in the intro, so could you explain in a bit more detail what marketing mix modeling is and how it differs from the kinds of research and reporting that brand managers are likely already receiving from their marketing teams or agency partners?
Greg Dolan: So there’s a big difference between what was traditionally marketing mix consulting and what we do. In the past – and again, I was a consumer of the traditional marketing mix – every single year, you would go through an exercise with a consultant where you would deliver to them your financial data, all of your marketing activity data across your marketing mix. So your TV data, radio, all your digital, your trade for the CPG world. And those consultants would build a custom analytics model, primarily time series regression. So they’re looking at the correlation between all of your activity and spend and your sales. And they would deliver that analysis in a PowerPoint presentation that gave you a report card for how the brand or business had done over the past two years. And that is an exercise that’s recreated and basically, done over every single year, created from scratch. So it was helpful in terms of, “Hey, I have an understanding of what worked in the past,” but it wasn’t, as I mentioned, as you know, helpful when it came to making the next decision and understanding what you do next. And that’s really what we are looking to disrupt. So the brand manager in the past would use that as validation for what happened in the past. What we’re trying to do is really pivot that from measurement to decision-making. So we know in this world where it’s a lot more complex, there are a lot more channels, a lot of silos within organizations that it’s important to have an omnichannel perspective when it comes to planning your marketing. So we are focused on bringing in all the information that we can bring to bear. So that’s a combination of the traditional time series data that you would give to a traditional marketing mix, but combine that with industry knowledge through our proprietary marketing elasticity engine, which serves as an information base for our models, but also any other analytics that is being done across the organization, whether that’s MTA, or copy effectiveness studies, or A/B tests or any of that information that can also inform the model. So we’re bringing the full knowledge state to the table in order to inform not only what happened in the past but, more importantly, on a real-time basis. “How do I make the next decision? Where am I going to get the most significant return on the next dollar invested? And how do I show continuous improvement for the brand looking forward?” So the use case there for both marketing teams and agency partners is that they’re using this as a planning tool that they can understand and simulate outcomes and then execute those plans and be able to validate the original plans through the system. So it’s a continuous planning, execution, and validation system.
Adrian Tennant: What typically prompts brand marketers to seek out a marketing mix modeling solution, do you think?
Greg Dolan: Well, it’s becoming a lot more complex to understand all the different pieces of marketing, and how do you balance the top of the funnel, mid-funnel, and bottom of the funnel? I think what we’ve seen is that, you know, where we’ve had the best luck understanding impact is at the bottom of the funnel. And, you know, those revenue-driving transactions. So, what we know is that there’s a lot of complexity, a lot of fragmentation across the funnel. It’s as important to have a balance at the top of the funnel as it is to have a heavy investment at the bottom of the funnel. So brand managers and marketers, in general, are looking to understand the full impact of the marketing mix on financial performance. And that’s been largely something that marketers haven’t been able to demonstrate. And that’s one of the reasons why, You know, CMOs have a very, uh, short tenure in the C-suite. They’re not able to demonstrate with accountability the impact of the decisions that they’re making across the marketing mix in service of those financial objectives they’re looking to achieve. Really closing that marketing proof gap, being able to understand, “I’m putting a dollar in all these channels, what does it mean for the value that I’m driving for the organization as well as the short-term impact from a revenue perspective?”.
Adrian Tennant: What are some of the most common use cases for MMM?
Greg Dolan: The primary use case – and it’s a little bit different for the way we approach it. So we approach it from a decision-making perspective, and we’re working with clients and agencies to develop a decision frame around what decisions they’re looking to make. And the best way to think about that is in terms of a hierarchy. So if I’m the CMO of an organization and I have a lot of different brands across my portfolio, the first decision I want to make is understanding, “How much do I put behind brand A versus brand B?” And then, we can get to lower levels of granularity. So, for brand A, maybe I want to understand sub-brand A versus sub-brand B and then across all the channels within each one of those brands. So it becomes a deeper and deeper understanding of how all of the marketing pieces, all the marketing channels, are contributing to the financial outcomes. And that’s really the primary use case for us: in a brand-based, macro-down perspective, around marketing mix from an omnichannel perspective. But then we can get to more granular use cases. So an example would be, a huge use case in CPG right now is understanding retail media and how that plays, you know, across retailers in interaction effects with shopper marketing and trade. And how potential retail media investment in, say, a Walmart impacts Target and other retailers. So we’re able to build models at the level where decisions are being made and give unique perspective on how to optimize those resources.
Adrian Tennant: Marketing mix modeling has traditionally been offered by large well-known marketing and shopper measurement companies, and it can often be several months before clients are presented with a model. These engagements also typically require a six-figure investment. Greg, your disruptive approach considerably compresses the traditional timeline and reduces the cost with a system designed to provide real-time insights. How do you achieve that?
Greg Dolan: Well, the secret is software. So we realized early on that the market was moving a lot faster then a human could actually inform decisions. Back when I started my marketing career in the late nineties, you know, I had a decide between tv, radio, and print, right? So there were a couple of channels, we could take the time. There were long lead-time buys, right? A lot of upfronts. And we were able to do that, and it was perfectly fine to have, you know, a report that was provided once a year. And as digital became more prevalent and that fragmentation started happening, and then we got into shopper marketing on the CPG side. And then there’s retail media. There’s more and more fragmentation, and the buying cycles are shorter. So you can’t make decisions or understand impact without technology and without the right amount of data to be able to support that. And if you’re looking at a pure consulting model, it’s cost prohibitive because each model is very expensive, to your point, north of six figures. So technology had to drive efficiency there. So we were able to build a model that leverages information, ingests and maps information in real-time, builds models with the click of a button. And then you have a historical perspective that’s based on the latest information and a foundation then to run planning optimizations and look forward and start to build, you know, prescriptive plans based on the latest information, based on a learning model that embeds machine learning and AI into that decision-making process. So really all enabled by technology, and the ability to quickly ingest and map data, when it’s available into something that’s actionable that the marketer can though go execute.
Adrian Tennant: Keen recently published details of a study that reveals the most effective flighting strategy for linear TV advertising. Greg, could you share with us what you learned?
Greg Dolan: Absolutely. It’s fundamental to the math in our system. So as I was talking about before, you know, we’ve accounted for long-term effects in our models. So in our marketing elasticity engine, we have different decay rates for every single marketing channel you can think of – so for TV versus radio, print versus couponing, and some of the shorter transaction-driving tactics as well. So we’ve built, based on that, a response curve weekly into the future. So we know exactly, based on both internal and external factors, what the point of diminishing returns are for a given channel, including TV. So we can see what the marketer is giving up from a revenue and profitability standpoint by going dark, right? So if you’re flighting, and you’re two weeks on, two weeks off, or three weeks on, three weeks off, those three weeks that you’re off actually, there’s an opportunity cost that’s created where you’re giving up profitable demand. So we’ve been able to show the power of continuity, and the impact that has on building value over time. And as I was talking about before, when you are dark, it’s more costly to then get back on, get in front of consumers, and have that spend drive profitable demand in the short-term. So really it is all about driving continuity of activity as a means to continue to build, as marketing decays over time.
Adrian Tennant: Let’s take a short break. We’ll be right back after this message.
![]() | Lydia Michael: Hi there. I’m Lydia Michael, the author of Brand Love: Building Strong Consumer Brand Connections. Reflecting my experience as a multicultural marketing and brand strategist, Brand Love is for any marketing and brand professionals, entrepreneurs, and those who oversee brand messaging, communications, and other consumer-facing strategies. Whether you work for a big or small brand, the book is designed to provide you with actionable strategies to grow and build any successful brand. As an IN CLEAR FOCUS listener, you can save 25 percent on a print or electronic version of Brand Love by using the exclusive promo code BIGEYE25. This code is valid for all products and pre-orders and applies to Kogan Page’s free paperback and e-book bundle offer. When you order directly from Kogan Page, shipping is always free to the US and the UK, so to order your copy of Brand Love go to koganpage.com. And thank you! |
Adrian Tennant: Welcome back. I’m talking with Greg Dolan, the co-founder and CEO of Keen Decision Systems. Artificial intelligence is currently very much in the news, but at Keen Decision Systems, you’ve been using it for several years. Greg, how does the inclusion of machine learning impact your platform’s predictive and prescriptive planning outputs?
Greg Dolan: So we think about ourselves as an AI decision-support agent, right? So the system is based on the fact that the model will learn from new information as it’s ingested in the model, right? As we were talking about before, old-fashioned, more traditional marketing mix is a point-in-time analytics exercise that’s recreated every year. So there’s no learning from the new information. It’s actually a new analysis. Ours is actually built and additive over time. So our model is based on, you know, thousands of data points over time from our marketing elasticity engine that’s built on with every new model that’s published in the application. So marketers using our system are learning from the rest of the industry as they go, but also new data that they ingest that’s their own as part of their custom models. So every single data point that comes into the model teaches the model based on that data point. So the marketer is constantly making decisions based on the newest, most recent information.
Adrian Tennant: Well, you’ve mentioned it a couple of times: a patent-pending feature of your platform is the marketing elasticity engine. Greg, can you explain what that is and how it supports the optimization of marketing investments across different channels?
Greg Dolan: So it’s the best industry information possible. So we built our models based on Bayesian regression, which means that there’s prior information that becomes a starting point for all of our models. So we’ve built this database that’s initially populated with about 40 years of academic research. How much does a GRP for TV deliver on average for typical brands and businesses across industries? We’ve then built in this automated mechanism where every single elasticity is blinded and informs the elasticity engine. So, when we publish a model, we learn from the changes in the data patterns and signals for businesses across industries. It helps to infuse the marketing mix model with more information, more relevant information, we’re able to source from businesses across industries. So it actually makes the model, you know, more predictive and more prescriptive over time. The other aspect I’ll just add is that, we can use that information to simulate marketing channels that have not been executed in the past. So if the brand is considering as an example, investing in a new social media platform, we will have an elasticity in our engine that can form that initial investment. So you can actually simulate, if you’re looking to optimize a fixed budget, you can simulate the allocation for a new channel, and we could see exactly where we’d pull those dollars to be able to fund that new channel.
Adrian Tennant: So that’s very powerful – if a client is considering adding a new channel to the media mix but has no prior data of their own, Keen Decision Systems’ model can predict their performance.
Greg Dolan: Correct. And then, as we get experience in that channel, so we execute the spend and we then incorporate that new data in the model, the model will adjust based on that data signal, and the actuals coming out of the market.
Adrian Tennant: Does your model typically allow for factors such as pricing changes, new distribution partners, the broader economic environment such as inflationary pressures, and seasonality?
Greg Dolan: All of the above. So you can’t really forecast the right investment plan or forecast, you know, the top and bottom line without a good understanding of the external factors that are impacting your business. So we incorporate all of those into the model, and their key factors that contribute to what the right amount of spend is and what the impact of that spend is across channels. You know, a good example obviously, is COVID. I mean, as we went in, we really leaned in with our clients, with all that uncertainty, incorporated a hundred-year pandemic into the models. So we were able to see in real-time as the pandemic unfolded, you know, how behavior was changing, how channel performance changed, and we were able to make changes with a lot of agility through that time period that, you know, helped the brands grow even in the face of all that adversity.
Adrian Tennant: What types of data integrations does your platform support?
Greg Dolan: This was always historically the biggest challenge for marketers. With marketing mixes, you know, the heavy lift associated with collecting data. So we’ve spent the last, you know, 10-plus years really focused on the data problem and have as much flexibility in ingesting data as possible. So we have APIs, so we’re able to connect into data systems. We have data connectors that are connected in, so all of your major platforms can connect directly into our system. And in the absence of that, we’ve developed some CSV templates that can be automatically uploaded and mapped. And we have smart mapping within our application, so based on how we’re modeling and at what level we’re bringing the right amount of data into the system to quickly model and build plans.
Adrian Tennant: How frequently are Keen Decision Systems’ models updated?
Greg Dolan: So it depends on the client’s decision cycles, as we call them. You know, how often are they looking at marketing and understanding marketing performance and then making any changes to marketing? So the system is set up to update every single time there’s new data. But on average, we see clients that are updating quarterly. Some of our clients are as they get more accustomed to the model and leverage it more their decision-making have moved to monthly, which also then can inform their monthly forecasts, for the business as well. So as often as, new information is available, they can update them. But it also depends on when the information will be utilized for decision-making.
Adrian Tennant: Typically, what’s the margin of error for revenue forecasts generated by your system?
Greg Dolan: So generally, it starts pretty accurately. As I was mentioning before, we use a lot of data, a lot more data than just time series data to inform our models. So a combination of the marketing elasticity, priors, as well as other analytics, as well as the time series and financial data, actually leads to more prescriptive and predictive models. So on average, to start, we’re less than 4% error. And as the model learns more over time, through the course of our relationship with brands and businesses, that will tighten even further. And the more we get to know the business and can incorporate the right information, that will tighten as well.
Adrian Tennant: Greg, could you walk us through some case studies that illustrate your platform’s capabilities?
Greg Dolan: Sure. I mean, we work with a pretty diverse group of clients. So we’ve built the system to be industry and data-agnostic. So, you know, we’ve worked with clients anywhere from direct-to-consumer cleaning products, to golf grips, to food delivery systems, right? So there’s a lot of different brands we work with. The primary use case really is around financial optimization. So, you know, on the DTC cleaning products, we really wanted to get to a monthly optimization cycle with them using the platform to refresh data channel optimization, timing optimization. And for that brand in particular, and this is the key that I’ll mention across all the case studies I’m going to talk about, we’re able to identify pretty sizable financial opportunities. And in this case, we’re able to identify a $33 million top-line opportunity. So there are real dollars and cents once you understand where the waste is, to be able to reallocate and optimize. You know, I mentioned the frozen food delivery company that we work with. You know, they had some challenges from COVID. During COVID, you know, a lot fewer people were going to restaurants, a lot more people were getting food delivered to them. So their business really peaked as they went through 2020, but then had to cycle it in 2021. So our role changed from ‘20 to ‘21, where we’re helping them to fuel the growth in 2020, but then had to identify how to cut back in 2021, right-size the investment to make sure that we’re still optimizing the ROI, and able to drive some growth even though there were external headwinds. And then, you know, the example with golf grips, right? They were looking to, one, get a lot more impact with the same budget. So we’re able to show them a path to optimize both their timing and their mix of spend across channels to drive – and I know it’s going to sound crazy – but a 344% increase in ROI increased revenue by $14 million. So real dollars and cents, real value being driven, just knowing in real-time where the dollars are most effective and where you can step on the gas and invest more.
Adrian Tennant: Over the past several weeks on this podcast, we’ve been examining how brands grow, discussing some of the universal laws established by the work of the Ehrenberg-Bass Institute. Greg, how does Keen’s analysis reflect or support Ehrenberg-Bass’s principles?
Greg Dolan: So we talked a little bit about continuity of spend and I think, paramount to Byron Sharp and How Brands Grow is making sure that you’re constantly in front of consumers, and as broad reach as possible. And I think that’s what we’ve scientifically proven out as well. So you can’t be dark. You have to be in front of consumers. You have to maintain continuity and consistency in your spend to be able to continue to drive growth in the brands. And we, given that we have that long-term effects viewpoint of how businesses grow, we’re actually able to show the layers of rock. And we have a chart in our system that show layers of rock and how marketing year after year is building value over time. And that’s very consistent with what Ehrenberg-Bass would promote as well.
Adrian Tennant: If IN CLEAR FOCUS listeners are interested in learning more about marketing mix modeling with Keen Decision Systems, what’s the best way to get in touch with your team?
Greg Dolan: Please reach out via our website, www.keendss.com and you can request a demo or an introductory conversation, and we’d love to share more.
Adrian Tennant: And if you’d like to see some of the platform’s capabilities, follow the link to the short video included in the transcript for this episode. Greg, thank you very much for being our guest this week on IN CLEAR FOCUS!
Greg Dolan: Thank you, Adrian, I really enjoyed it. And again, we’d love to have a conversation with anyone that’s interested in more background on Keen.
Adrian Tennant: Thanks again to my guest this week, Greg Dolan, the co-founder and CEO of Keen Decision Systems. As always, you’ll find a full transcript of our conversation along with links to the resources we discussed on the Bigeye website at Bigeyeagency.com. Just select podcast from the menu. Thank you for listening to IN CLEAR FOCUS, produced by Bigeye. I’ve been your host, Adrian Tennant. Until next week, goodbye.